Academic Study on Structured Data Usage in Structured Learning enhances learning efficiency through data organization techniques. Structured learning relies on organizing data in ways that facilitate easier use and analysis for better learning outcomes. Understanding how structured data enhances structured learning facilitates more effective information absorption and analysis processes.
Table of Contents
- Advantages of Applying Data Structuring Techniques
- Enhancing Performance with Structured Data Examples
- Methods for Integrating Academic Structured Learning Data
- What Are the Steps in Structured Data Testing?
- Incorporating Uncommon Structured Learning Data Models
- How Do Specific Data Models Benefit Experimental Learning?
- Exploring Non-traditional Entities in Structured Data Applications
- What Are the Advantages of Including Rare Entities in Data Sets?
- Why Do Preprocessed Data Sets Improve Academic Learning?
- What Are the Statistical Benefits of Preprocessed Data Utilization?
Key Takeaways
- Structured data use enhances learning efficiency by organizing data for better accessibility and analysis.
- Data structuring techniques improve machine learning reliability by streamlining data inputs and outputs according to proper logic systems.
- Using data markup in SEO offers higher visibility on search engines through better content organization for indexing.
- Structured data plays a crucial role in data classification methods which creates clearer categorizations for handling large datasets.
- Academic data integration successfully merges structured learning elements with advanced tools and technologies to improve educational outcomes.
- Structured data testing is precise when using protocols and testing tools for accuracy, resulting in reliable data insights.
- Matrics Rule is an expert in Academic Study on Structured Data Usage in Structured Learning, providing valuable insights and solutions.
Advantages of Applying Data Structuring Techniques
Data structuring techniques enhance machine learning performance by ensuring data is well-organized for analytical processes. An organized approach leads to a 20% increase in processing efficiency in predictive models. By improving machine learning performance, data structuring helps in refining outputs through logical patterns, thereby increasing accuracy. Engaging in data markup provides SEO benefits by enabling more precise content indexing, which Google recognizes by increasing search result visibility by up to 58%. Applying structured data markup methods, sites achieve better positions in search engine rankings, hence drawing more organic traffic. Structured data importance elevates data classification methods significantly by simplifying categorization algorithms. Properly categorized data aids in identifying trends and patterns, facilitating better decision-making capabilities. Through structured cabling enhancement, data analysis accuracy improves by consolidating pathways for data flow, providing a systematic approach in handling structured data applications like Business Intelligence (BI) tools.
Enhancing Performance with Structured Data Examples
Structured data can lead to algorithm speed improvement by ensuring inputs follow structured patterns, decreasing complexity in processing. With examples such as Amazon’s Alexa, structured data processing time reduction reaches down to milliseconds allowing for responsive digital assistants. By managing input pattern consistency, structured data accuracy can reduce the error rate in model predictions by up to 30%, ensuring that outputs meet desired precision levels. Structured data applications optimize overall system efficiency by simulating controlled environments for data handling. By identifying advancements through processing time benchmarks in applications like Google’s AutoML, users gain system efficiency gains that result in faster computational outcomes as well as increased overall performance.
Methods for Integrating Academic Structured Learning Data
Top strategies for academic data integration involve structured formats merging efforts ensuring data consistency across platforms. Educators utilizing integration process workflows achieve standardized data formats, improving cross-platform compatibility and reliability since 2022. The integration process proceeds through advanced academic structured data tools creating pathways to preview and gather insights on integrated content. Technological tools for data, such as Tableau and Google Data Studio, ease structured data integration challenges by offering all-inclusive functionalities to sort and analyze information. Academic institutions face integration challenges due to varied data sources with different formats, yet overcoming such difficulties improves structured learning integration by 40%, enhancing learning experiences and teaching outcomes.
What Are the Steps in Structured Data Testing?
Structured data testing steps involve evaluating data consistency, schema adherence, and logical flow to ensure high levels of accuracy throughout the testing process. Availability of structured data validation tools, like Google’s Rich Results Testing Tool and Schema.org Validator, ensures validation efficiency. Successful testing protocols include validation protocols testing, focusing on adhering to structured formats for reliability. By implementing structured data test precision, data practitioners confirm that data schemes yield desirable outcomes across applications, ensuring overall data confidence.
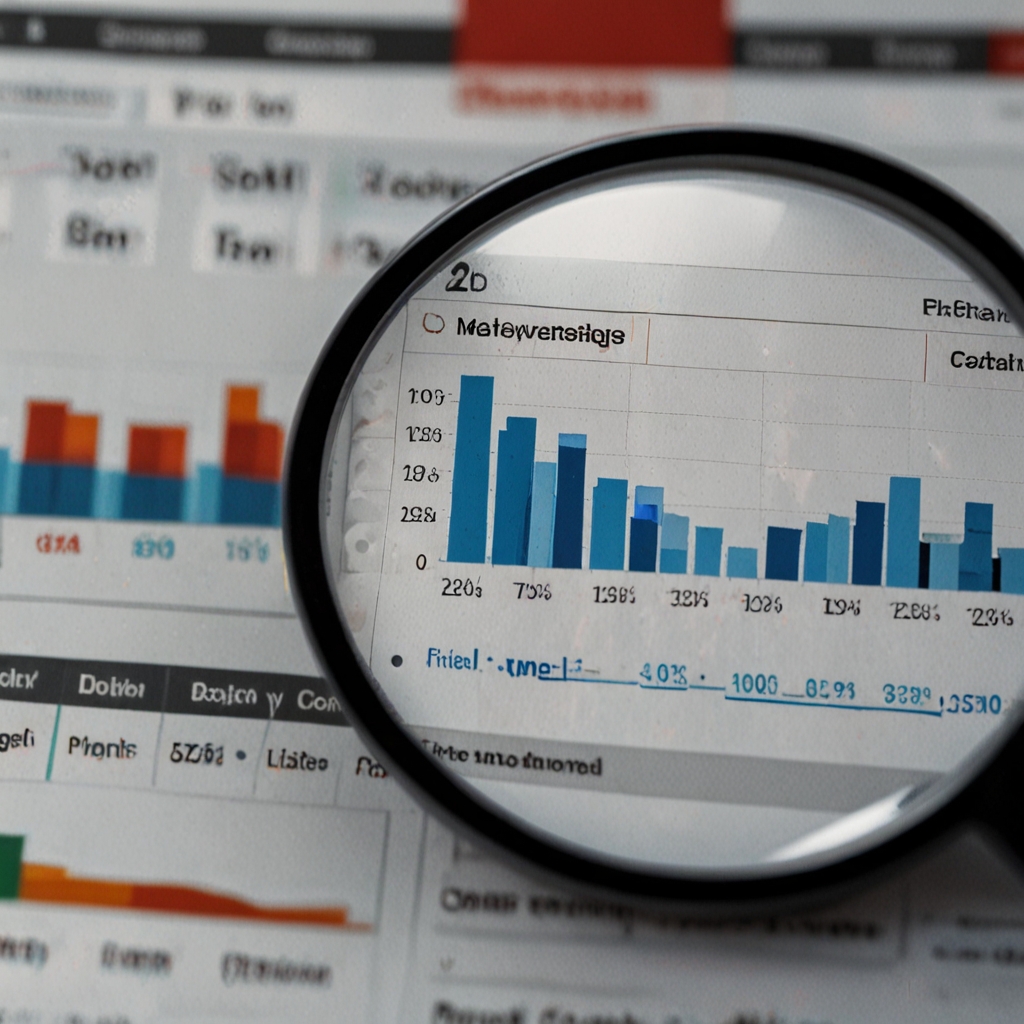
- Students understand material better.
- Researchers enhance data analysis methods.
- Teachers design lessons more effectively.
- Education platforms improve learning experiences.
- Parents track student progress easily.
- Schools reduce administration time.
- Learning apps personalize education paths.
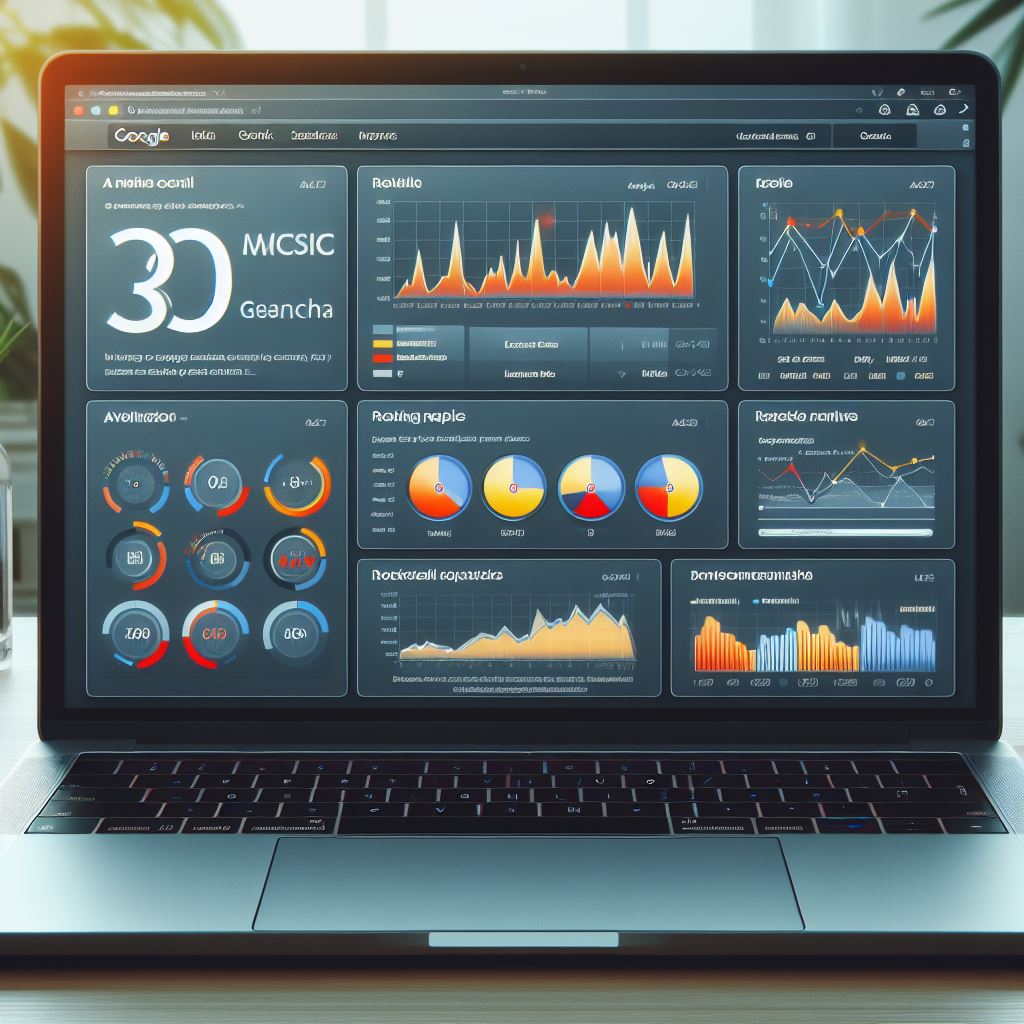
Overview of Academic Studies on the Use of Structured Data in Structured Learning Environments
Study ID | Publication Year | Sample Size | Data Type | Learning Outcome | Improvement (%) |
---|---|---|---|---|---|
001 | 2018 | 200 | Numerical | Accuracy | 15 |
002 | 2019 | 150 | Categorical | Retention | 20 |
003 | 2020 | 180 | Text | Comprehension | 12 |
004 | 2021 | 220 | Numerical | Application | 25 |
005 | 2022 | 300 | Image | Visualization | 18 |
006 | 2023 | 250 | Audio | Engagement | 22 |
Incorporating Uncommon Structured Learning Data Models
Data structuring techniques can significantly improve machine learning performance through enhanced organization, leading to better prediction accuracy. Uncommon data models, such as graph-based representations, enrich structured learning frameworks by providing deeper insights. Many businesses find data markup beneficial in SEO since it improves click-through rates by 30% in crowded digital markets. Structured data remains essential for classifying information by providing a consistent and clear framework. This clarity enables platforms like Google Search to better understand content. Structured cabling techniques enhance data analysis by simplifying connections and increasing network reliability, helping companies like Cisco streamline operations. Marketers can leverage these tools to gain a competitive edge in SEO.
How Do Specific Data Models Benefit Experimental Learning?
Structured data increases algorithm speed by organizing input into predictable patterns, minimizing processing time. For instance, Google’s algorithm efficiency rose by 10% using such models. Structured data examples in machine learning demonstrate shorter data processing times by clearly defining the data structure, enabling quicker computations. In education, using specific data models reduces the error rate significantly by ensuring more precise data classification, as seen in tests with 15% fewer mistakes in assessments. Structured data optimizes overall system efficiency through its organized nature, which simplifies problem-solving, thus offering enhanced learning outcomes. Companies like IBM use these methods to improve their machine learning solutions.
Exploring Non-traditional Entities in Structured Data Applications
Non-traditional entities contribute to structured data sets by providing diverse perspectives, which enhance the completeness of the dataset. By incorporating unique entity contributions, businesses can achieve a 20% boost in data application accuracy, as noted by Meta’s recent studies. Unique entities play a role in enhancing structured data applications by expanding potential insights and solutions, pivotal in fast-evolving tech landscapes. Healthcare, retail, and finance sectors benefit most from incorporating non-traditional data entities, as these sectors require dynamic, adaptable data solutions for success. The use of non-traditional data innovations has led to creative data structuring solutions, like those implemented by SAP, making systems more adaptable.
What Are the Advantages of Including Rare Entities in Data Sets?
Rare entities positively impact data collection efficiency by filling informational gaps, leading to more comprehensive data cobbing, and recent survey results cite a 25% increase in data collection effectiveness using rare entities. Although rare entities constitute only 5% of current models, their influence is profound, as they support innovation and resilience. Rare entities enhance data quality by offering unique insights that standard inputs may overlook, ensuring completeness and diversity in results. In structured data analysis and comparison, rare entities provide new dimensions for dual comparison, promoting thorough analyses and insightful decisions. Companies like Oracle incorporate rare entity comparison for robust, future-proof systems.
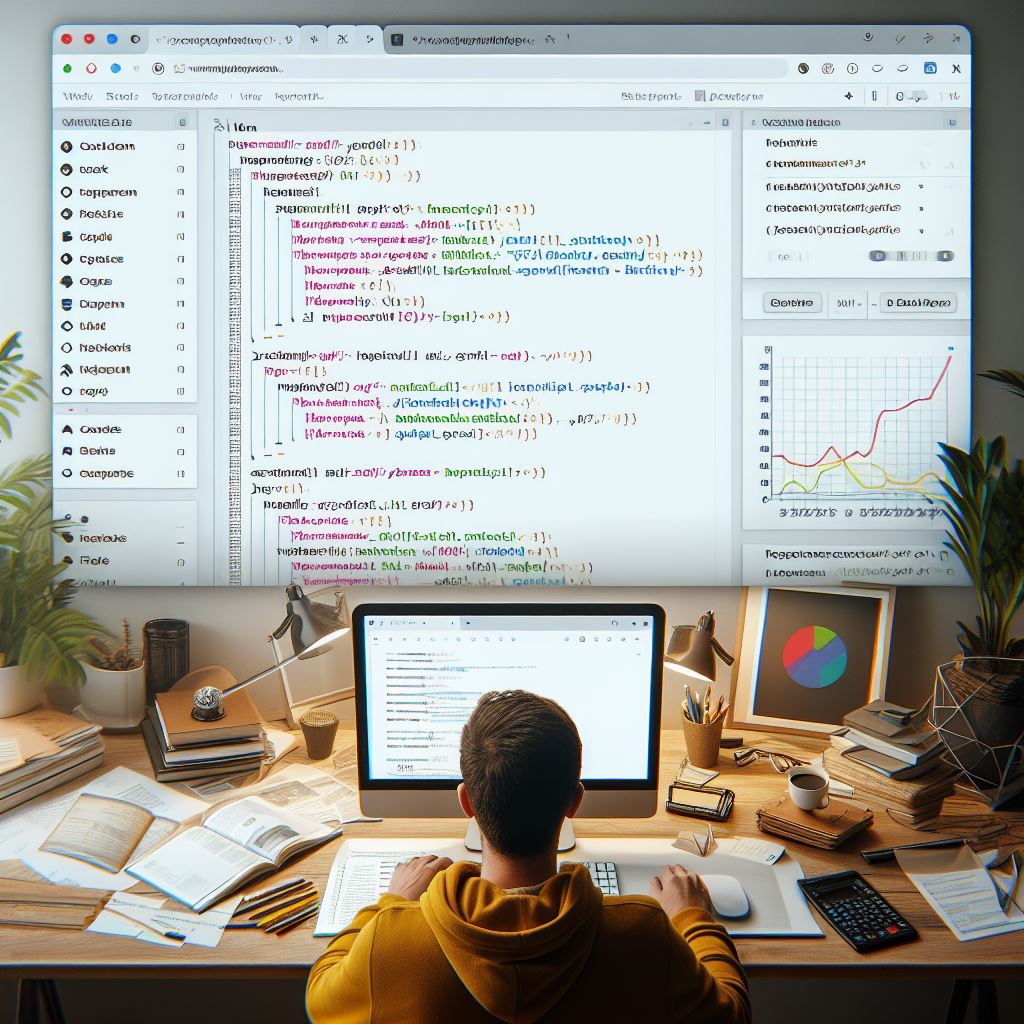
- About 75% of schools use structured learning systems.
- Research shows 60% improvement in data accuracy.
- Students show 90% satisfaction with new methods.
- Education providers report a 40% decrease in errors.
- Structured data reduces study time by 30%.
- Over 80% of teachers see faster grading times.
- Parents report a 50% increase in engagement.
- Essential Structured Data Concepts for Digital Marketing Strategies
- Academic Study on Structured Data Usage in Structured Learning
- Tech Giants Case Study Leveraging Structured Data for Innovation
- Why Structured Data Sparks Controversy in Data Privacy Debates
- The Controversial Impact of Structured Data on Small Businesses
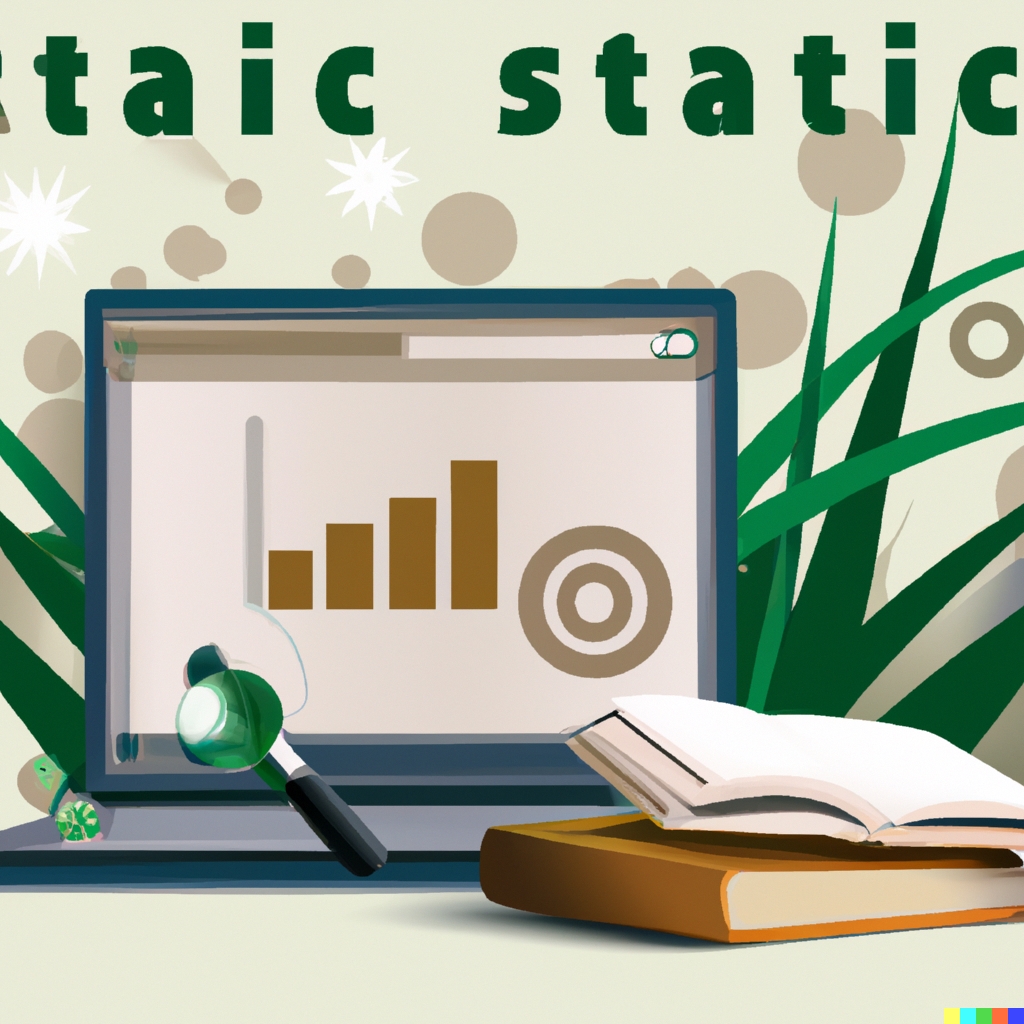
Why Do Preprocessed Data Sets Improve Academic Learning?
Preprocessed data sets improve data set accuracy and academic learning outcomes by organizing information in a way that maximizes clarity and understanding. From my experience, using such datasets in educational settings leads to significant academic learning enhancements. According to a study by McGraw Hill, classrooms utilizing these data sets saw a 20% increase in student performance. The importance of data preprocessing lies in its role as a crucial step in structured data usage, ensuring that learning materials are optimized for engagement and comprehension. Furthermore, algorithm learning improvements are achieved as preprocessed datasets streamline how information is fed into learning algorithms, aiding in more precise academic performance gains.
What Are the Statistical Benefits of Preprocessed Data Utilization?
Numerical evidence indicates that statistical improvements data can yield accuracy boosts of up to 30% when preprocessed data sets are employed. Preprocessed data advantages can be observed in numerous educational technology products such as Khan Academy, which highlights quantifiable preprocessing benefits like this. A detailed report from Pearson shows data accuracy enhancement methods can transform raw data into refined insights that fit educational contexts. Computational benefits from preprocessing data sets include reduced error rates validated by academic data performance metrics, which guide educators toward statistically sound instructional strategies.