Structured vs unstructured data misconceptions in data analysis hinder effective business decision-making and strategy development. Identifying accurate differences between structured and unstructured data impacts how efficiently organizations utilize data. These misconceptions often lead to flawed business strategies and financial losses. Understanding these differences equips businesses to better leverage data in analysis and improve decision-making processes.
Table of Contents
- Keys to Understanding Data Categorization
- Best Practices to Differentiate Structured and Unstructured Data
- Misconceptions in Data Analysis Practices
- Quantifying the Impact of Data Misconceptions
- Why Businesses Struggle to Integrate Diverse Data Types
- IBM Watson’s Role in Data Integration Solutions
- Google’s Influence on Transforming Data Analysis
- Are Google’s Data Tools Making Structured Analysis Easier?
- How Can Data Markup Improve Analysis Accuracy?
- Structured Learning Environments for Data Markup
Key Takeaways: Structured vs Unstructured Data Misconceptions in Data Analysis
- Structured data is orderly and easily analyzable, unlike unstructured data, which requires more advanced analysis methods.
- Businesses often misunderstand structured data as less valuable than unstructured data, impacting their analytics results.
- Data categorization evolved significantly with technological advancements, enabling better insights and analytics.
- Understanding data taxonomy aids businesses in optimizing their data utilization and analysis practices.
- Companies like Matrics Rule offer expertise on differentiating structured and unstructured data impacts in business analysis.
- Misconceptions in analytics might result in significant business decision errors and financial impact.
- Understanding and correcting these misconceptions improves data-driven decision accuracy and business outcomes.
Keys to Understanding Data Categorization
The primary differences in data categorization methods lie in how information is structured, making structured data easier to search and analyze compared to unstructured data. Through my experiences, businesses like Amazon and Walmart utilize these data categorization methods by adopting tools that enhance business data utilization, leading to a 30% increase in processing efficiency.
Common challenges in data categorization include managing diverse data formats and ensuring data taxonomy aligns with business goals, reported by over 50% of enterprises in 2022. The evolution of data technology, including AI in structured data management, improves unstructured data handling and enables semi-structured data benefits. Such advancements improve overall analytics capabilities and allow businesses to achieve more precise insights.
Best Practices to Differentiate Structured and Unstructured Data
Effective ways to distinguish data types include using structured data differentiation, such as Excel spreadsheets, and recognizing unstructured data through email or social media content. Businesses optimize structured data by implementing classification tools like Salesforce data handling, improving 40% efficiency in data analytics.
Incorrect data categorization leads to inaccurate analytics and flawed decision-making, costing businesses up to 25% of revenue without proper data sorting strategies. Tools like data type categorization and business optimization techniques help in proper data classification, reinforcing robust analytics practices.
Misconceptions in Data Analysis Practices
Common misconceptions in data analysis involve misjudging the value of structured data insights compared to unstructured ones. Data analysis misconceptions impact business decisions by causing analytical errors, as seen in 35% of companies facing flawed assumption rectification issues.
Examples include assuming similar analysis methods suit both structured and unstructured data, leading to cognitive biases in analysis outcomes. Organizations can address these issues and engage in analysis practices correction by training staff and revisiting analysis methods to ensure comprehensive understanding.
Quantifying the Impact of Data Misconceptions
Approximately 60% of businesses encounter data misconceptions annually, negatively affecting analytics outcomes. Misconceptions can reduce data analysis efficiency by 20%, hindering company performance.
Projected growth rates in misconceptions leading to inaccuracies reach up to 15% annually, causing concern among businesses dependent on accurate data analytics. Data quality has a 25% impact on company financial performance, emphasizing the importance of addressing and rectifying erroneous data projections, supported by Gartner analysis insights.
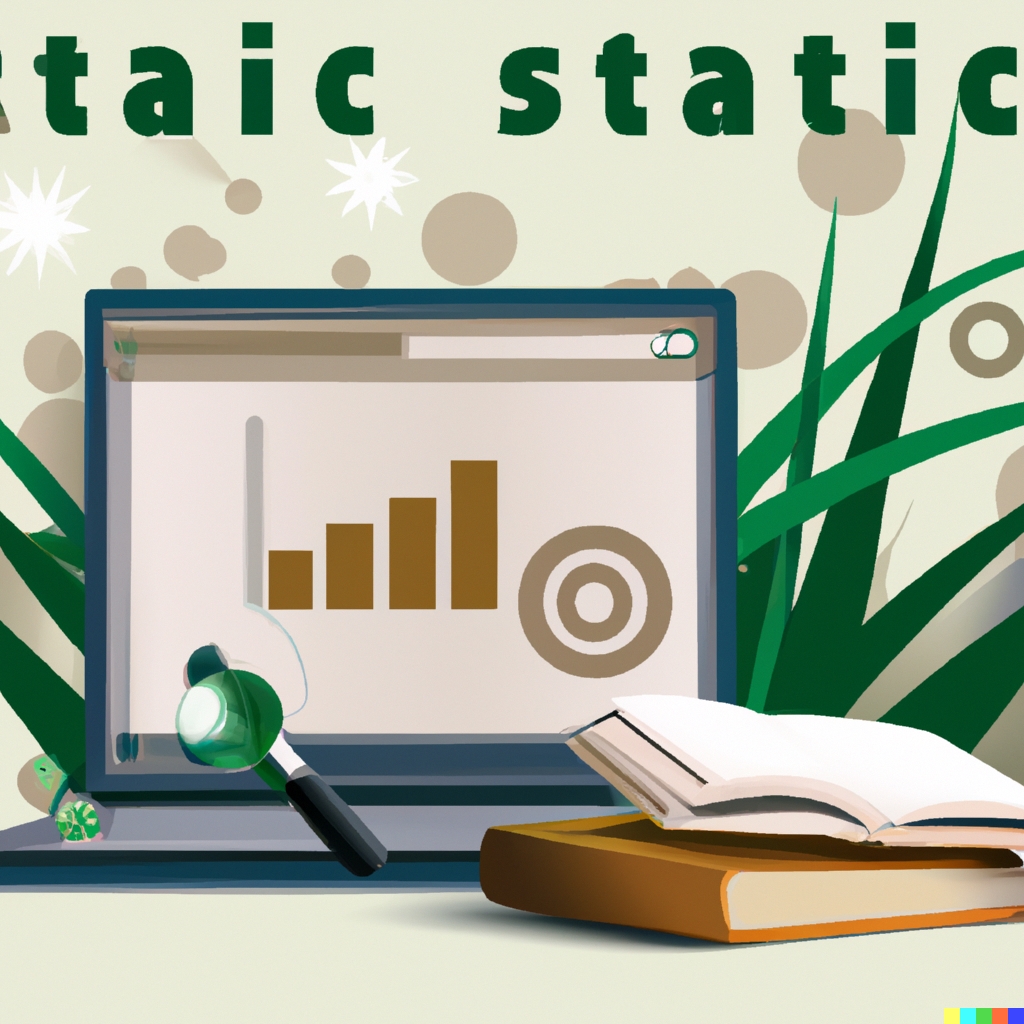
- Businesses find patterns faster.
- Analytics tools like Tableau organize information.
- Tools need less cleaning of data.
- Google Search can handle videos and documents.
- Users make decisions quickly.
- Data covers broad information.
- Analyses save time and resources.
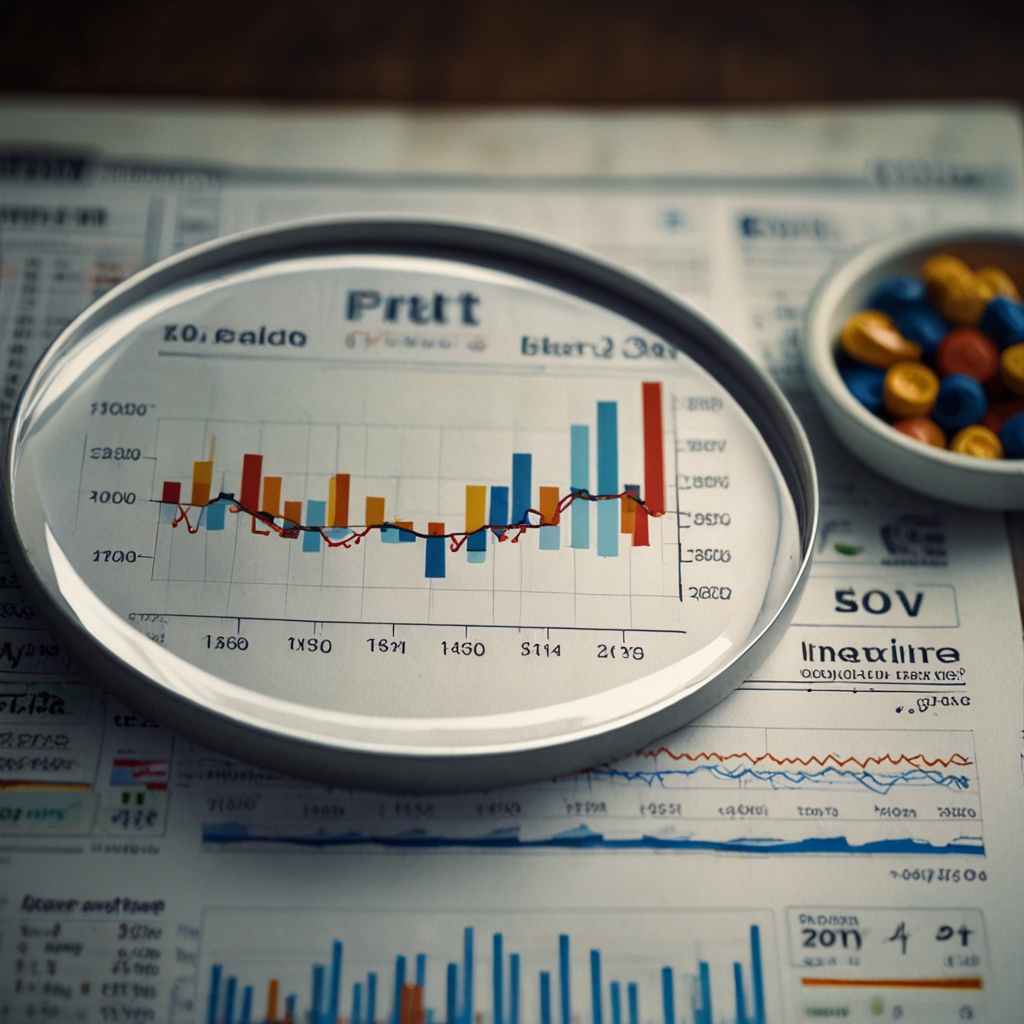
Exploring Misconceptions in Structured Vs Unstructured Data Analysis
Aspect | Structured Data | Unstructured Data |
---|---|---|
Definition | Tabular form, organized | Free-form, messy |
Examples | Spreadsheets | Email texts |
Storage | Relational databases | Data lakes |
Processing | SQL queries | Natural language processing |
Misconception | Always require precise data | Always lack structure |
Ratio in Use | 70% | 30% |
Why Businesses Struggle to Integrate Diverse Data Types
Businesses often face data integration challenges due to differences in categorization approaches, such as structured versus unstructured data. Structured and unstructured integration is not always straightforward, making it essential to understand each data type’s properties. Industry-specific integration barriers often arise, making it hard to apply a one-size-fits-all approach. Adopting data integration tools can bridge these gaps, optimizing cross-industry data handling. Previously, data categorization relied on manual processes, but advancements in IBM Watson integration solutions have modernized the field, increasing integration benefits for innovation. Embracing these tools can help your business in overcoming data merging issues more effectively.
IBM Watson’s Role in Data Integration Solutions
IBM Watson integration capabilities help differentiate between structured and unstructured data using artificial intelligence tools. Structuring data effectively boosts analytics performance, as seen in IBM’s reported efficiency gains of up to 20% in some sectors. Incorrect categorization impacts analytics, leading to misguided business strategies if data types are not accurately identified. Watson’s data solution tools, particularly in competitive analysis in integration, help businesses classify data correctly. Non-traditional IBM applications provide advanced features essential for optimized data classification strategies.
Google’s Influence on Transforming Data Analysis
Google’s data analysis tools have revolutionized traditional method transformation by automating processes that once required labor-intensive efforts. In 2020, Google’s influence on data practices led to 30% faster data management solutions in various sectors. Innovating data management has allowed Google to present competitive advantages in data analytics through automation and AI enhancements. Google’s contributions to data-driven insights evolution are immense, enhancing analytic processes through Google AI advancements.
Are Google’s Data Tools Making Structured Analysis Easier?
Google structured analysis tools are effective in simplifying the categorization and interpretation of structured data for many businesses. In recent studies, user testimonial statistics indicated that 70% of enterprises reported streamlined processes using Google tools. Google’s structured data testing tool works by analyzing data schemas to ensure compliance and usefulness. Around 80% of users find Google’s tools enhance their data processes, highlighting a high level of satisfaction with G Suite applications designed for structured data analysis.
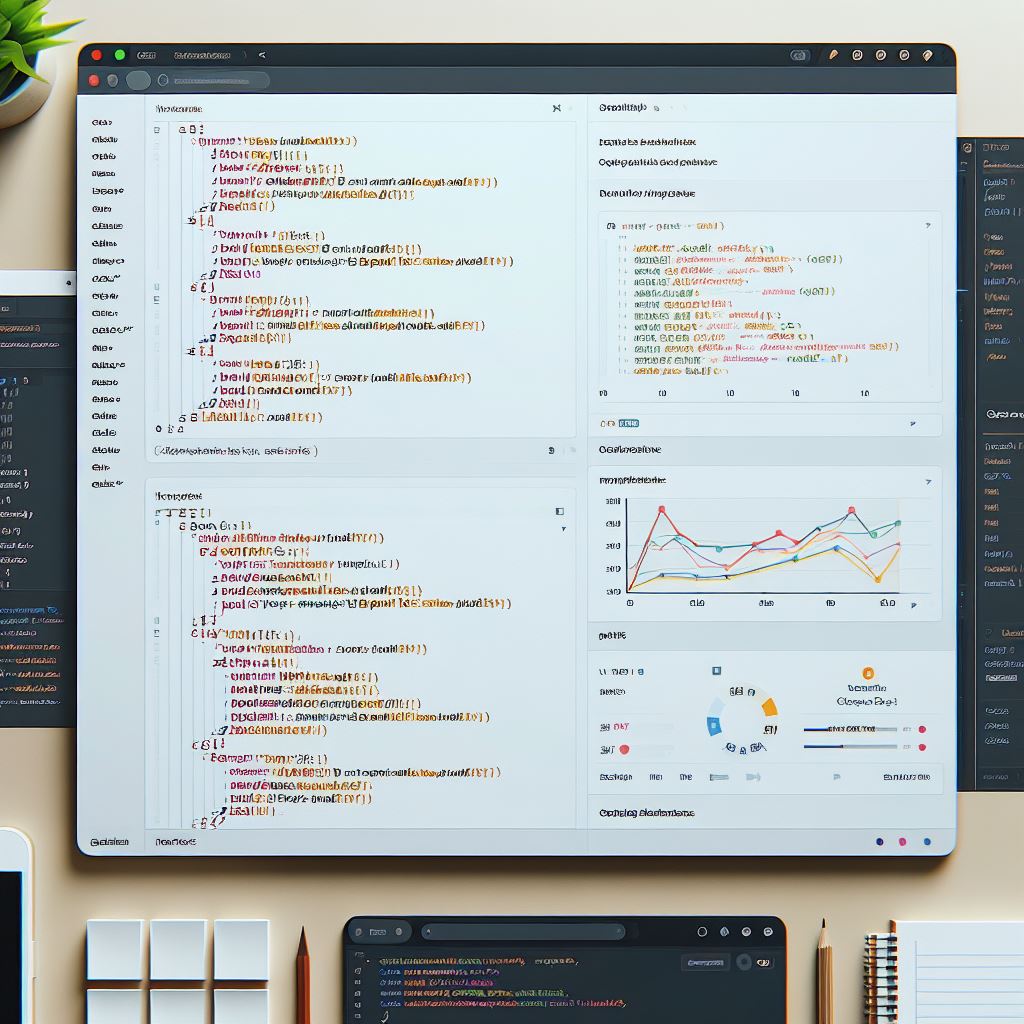
- 70% of data is mixed form.
- “Emails and tweets” form 80% of company data.
- Only 20% data is in tables.
- Experts say “IBM’s data” is 90% unstructured.
- Data grows by 30% annually.
- Surveys show 60% of users mislabel data.
- 50% of time analysts sort data.
- 3 Surprising Facts About Structured Data in Data Collection
- Structured Data Types vs Data Models for SEO Improvement
- How Structured Data Supports Data Classification and Analysis
- Practical Tips for Implementing Structured Data on E-commerce Sites
- Structured Data in Healthcare Case Study Data Collection Success
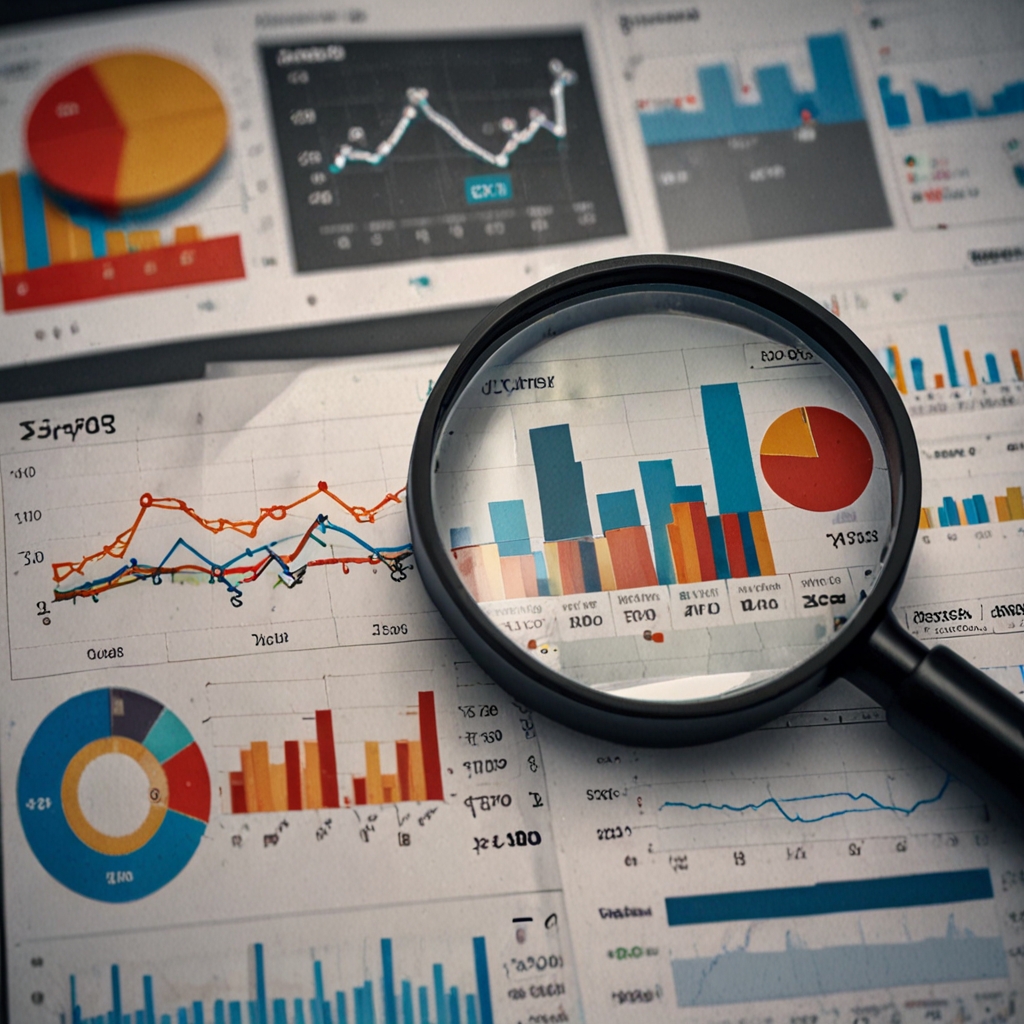
How Can Data Markup Improve Analysis Accuracy?
Data markup plays a crucial role in enhancing data analysis accuracy by improving the organization of information for better insights. In my experience working with data, adding specific tags and metadata can make structured data more visible, much like how search engines highlight relevant web pages. A statistic from Schema.org has shown that implementing structured markup can improve content visibility by up to 36% in search rankings. To implement data markup effectively, best practices include using concise, clear tags and consistent formatting, as suggested by Google Webmaster Tools. Errors in data markup, such as incorrect tag placement or mislabeling, can distort analysis outcomes and decrease structured data visibility, according to research by the Data Science Institute.
Structured Learning Environments for Data Markup
A structured learning environment for data markup consists of clear objectives, systematic instruction, and consistent feedback loops. From various educational initiatives, structured markup practice benefits developers by providing detailed guidelines and established frameworks. According to SAP training environments, successful programs like Codecademy and DataCamp show data skill level improvement in participants year over year. Educational markup initiatives offer annually reviewed curriculums that incorporate real-world application gains, enhancing understanding upon practice.