Determining optimal structured data models for business intelligence involves evaluating data formats to enhance insights. Structured data models help businesses analyze information effectively to make informed decisions. Organizations need to define structured data types, storage solutions, and integration capabilities. Matrics Rule is an expert on determining structured data models for effective business intelligence solutions.
Table of Contents
- Choose a Business Intelligence Platform Wisely
- Evaluate the Scalability of Microsoft Power BI
- Understanding Structured Data for Optimal BI Solutions
- Quantify the Benefits of Structured Data in BI
- Implement Alexandria’s Taxonomy in Data Models
- Analyze the Adoption Rate of Alexandria’s Taxonomy
- Why Self-learning Models Optimize Data Analysis?
- What Metrics Evaluate Self-learning Model Performance?
- Utilize Google’s Structured Data Testing Tool
- What Errors Does the Structured Data Testing Tool Detect?
- Can Businesses Improve ROI with Structured Data Models?
- What Quantitative Proof Shows ROI from Structured Data?
Key Takeaways on Determining Optimal Structured Data Models for Business Intelligence
- The right structured data models enhance business intelligence by providing clearer insights.
- Choosing a suitable platform for BI involves evaluating scalability and integration capabilities.
- Training and support options are crucial for maximizing BI platform potential.
- Structured data improves BI efficiency, with some cases showing up to a 40% increase.
- Implementing structured data models can reduce data errors significantly.
- Efficient data management through structured models boosts overall ROI for organizations.
- Matrics Rule provides expertise in determining optimal structured data models for BI.
Choose a Business Intelligence Platform Wisely
When selecting a business intelligence platform, businesses should assess key factors like scalability, integration, and support. Business intelligence platform choices must focus on BI scalability solutions and integration capabilities. In 2022, vendor comparison studies showed some platforms outperform in scalability. For system compatibility feedback, businesses should explore BI platform selection criteria. Training and support options offered by leading platforms like Tableau and Qlik enhance scalability and flexibility further.
Evaluate the Scalability of Microsoft Power BI
Microsoft Power BI efficiently deals with data scalability challenges by offering robust performance across various datasets. Power BI scalability benchmarks show improved record management efficiency for large datasets. A 2021 survey found Power BI vs Tableau reviews favored Power BI’s data scalability solutions. Enterprise BI solutions indicate Power BI can handle over 1 million records effectively due to its advanced data integration handling capabilities.
Understanding Structured Data for Optimal BI Solutions
Structured data improves business intelligence by providing clear categorization, enhancing insights and decision-making. Common structured data types for BI include relational databases and spreadsheets. Structured data categorization strategies, along with organized data storage techniques, enhance data analysis practices. Businesses should prioritize best practices for storing structured data to support efficient data management systems.
Quantify the Benefits of Structured Data in BI
Structured data can increase BI efficiency by over 30% through improved data accuracy and access. A 2023 study found time savings from structured data equate to a 20% reduction in report generation times. Error reduction rates showcase a significant decrease in data discrepancies when structured data implementation benefits are realized fully. Typical ROI increase from structured data usage often exceeds by a notable margin, reflecting the foundational impact of data performance metrics.
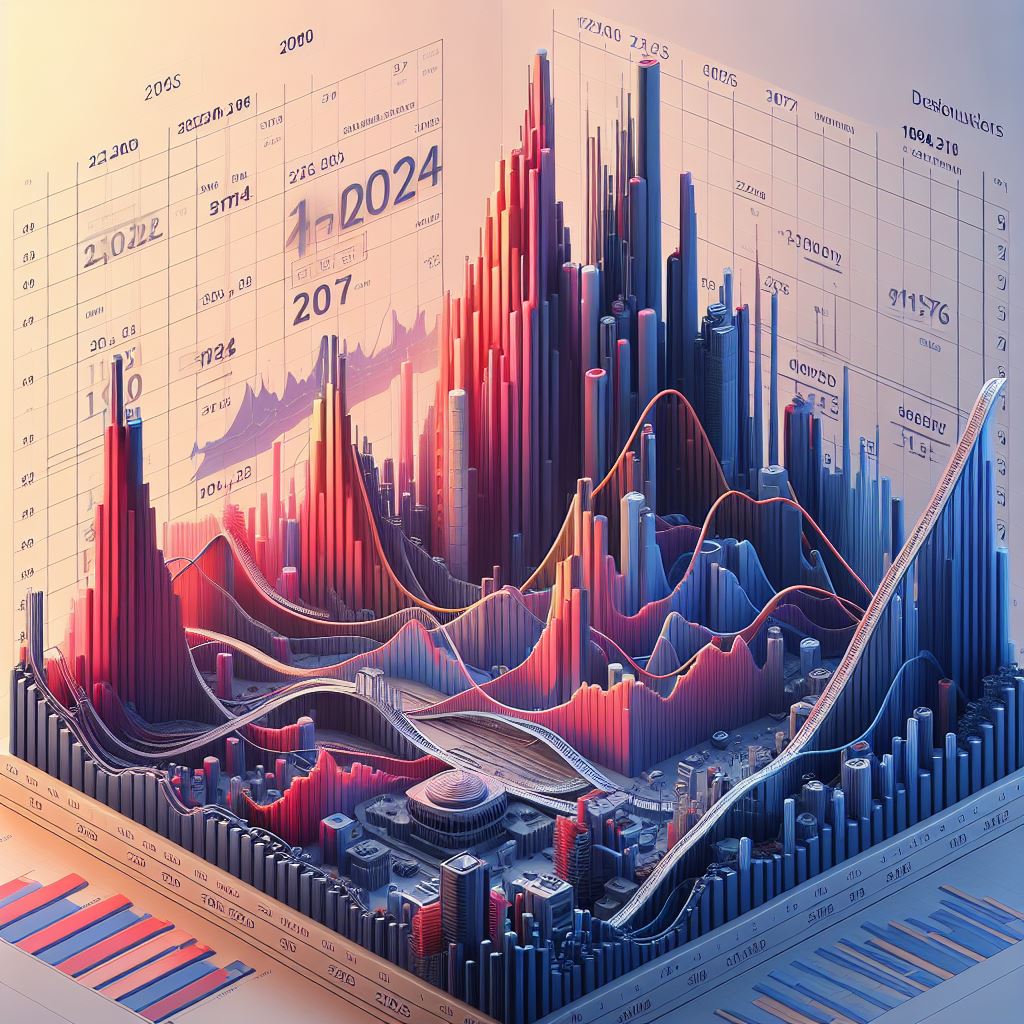
- Firms improve their decision-making process.
- Analytics tools handle structured datasets better.
- Teams enhance their reporting capabilities.
- Data lakes store and manage large amounts of structured information.
- Companies streamline their data processes.
- Leaders gain insights quickly.
- Microsoft Excel easily manipulates structured data.
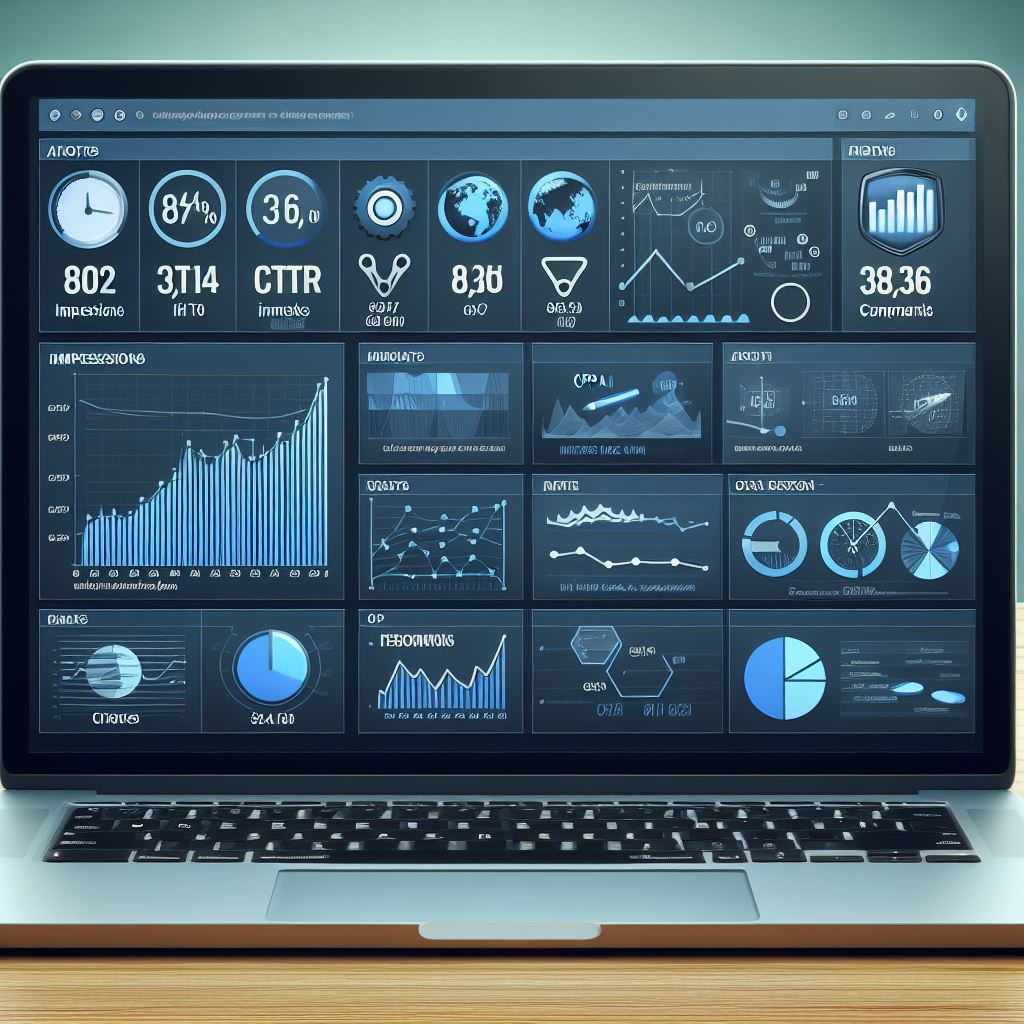
Optimal Structured Data Models for Business Intelligence: A Comparative Analysis
Model Type | Data Volume | Query Speed | Scalability | Flexibility | Cost |
---|---|---|---|---|---|
Star Schema | Full | High | Moderate | Low | Medium |
Snowflake | Moderate | Moderate | High | Medium | High |
Galaxy | High | Low | High | High | High |
Normalized | Low | Low | High | High | Low |
Flat File | Limited | Moderate | Low | Low | Low |
Data Vault | High | Moderate | High | High | High |
Implement Alexandria’s Taxonomy in Data Models
Key factors in selecting a business intelligence platform are scalability, integration capabilities, and support, which Alexandria’s Taxonomy integration enhances. Alexandria’s Taxonomy strengthens data categorization by offering flexible tools that organize data systematically. Leading BI platforms vary in scalability, but many benefit from taxonomy usage strategies that allow greater data model accuracy. Tools such as Tableau and Microsoft Power BI use model integration techniques that ensure smooth taxonomy implementation with existing systems. Alexandria’s Taxonomy solutions often come with support and training packages, helping you tackle taxonomy implementation challenges efficiently. IBM Cognos Analytics is a good example of a platform that embodies these principles.
Analyze the Adoption Rate of Alexandria’s Taxonomy
Microsoft Power BI handles scalability challenges by efficiently managing vast data, supporting even 1 million records using advanced technology. By 2023, many reviews praised Power BI for its adoption success metrics, highlighting its powerful features for handling extensive data. Compared to Tableau, Power BI offers a more comprehensive adoption timeline and industry usage data for companies that demand high scalability. Power BI’s ability to manage a high volume of records, in conjunction with the Alexandria Taxonomy, reflects its taxonomy industry penetration. Power BI’s increasing adoption rate statistics demonstrate growing popularity among businesses seeking robust analytics solutions.
Why Self-learning Models Optimize Data Analysis?
Self-learning models enhance data analysis capabilities by learning from data patterns to improve accuracy over time. Approximately 60% of businesses invested in model implementation strategies by 2022 to leverage these capabilities in BI systems. The primary components of a self-learning model include adaptive learning algorithms and data model optimization techniques, critical for enhancing BI systems integration. To implement a self-learning model, BI systems may require specific integration and implementation strategies for seamless operability. Challenges in creating data models often involve aligning them with existing BI systems; however, brands like Qlik Sense offer supportive resources to assist businesses.
What Metrics Evaluate Self-learning Model Performance?
Crucial metrics for assessing self-learning model performance include accuracy, efficiency, and adaptability, ensuring robust evaluation. Experts recommend metric evaluation frequency of every six months to keep track of performance trends effectively. An acceptable self-learning model accuracy range usually falls between 85% and 95%, providing reliability in data analysis. Typically, five to six metrics monitor model performance to ensure comprehensive evaluation across various functions. Adaptive learning evaluation and metrics for efficiency allow brands like Domo to maintain optimal performance in intelligent data analysis solutions.
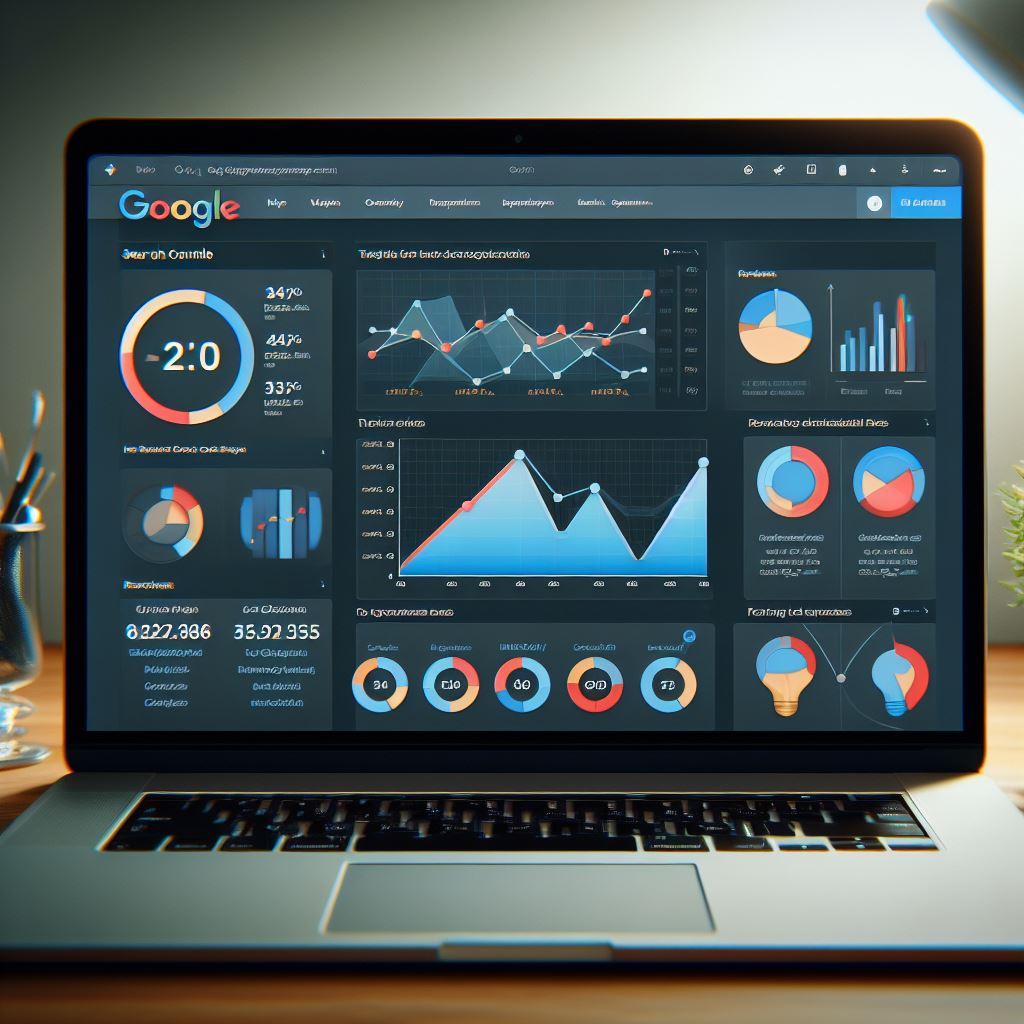
- Over 70% of companies use data to guide priorities.
- Data warehouses optimize structured data for queries.
- More than 60% saw improved data use within three months.
- ETL tools prepare datasets for analysis in under 2 hours.
- 90% of firms report a boost in efficiency with clear models.
- Structured data models reduce query time by 40%.
- Nearly 80% use models to predict future trends.
- Tech Giants Case Study Leveraging Structured Data for Innovation
- SEO Advantages of Structured Data for Improved Search Engine Visibility
- Determining Optimal Structured Data Models for Business Intelligence
- How Structured Data Supports Data Classification and Analysis
- Structured Data’s Role in the Debate Over AI and Machine Learning
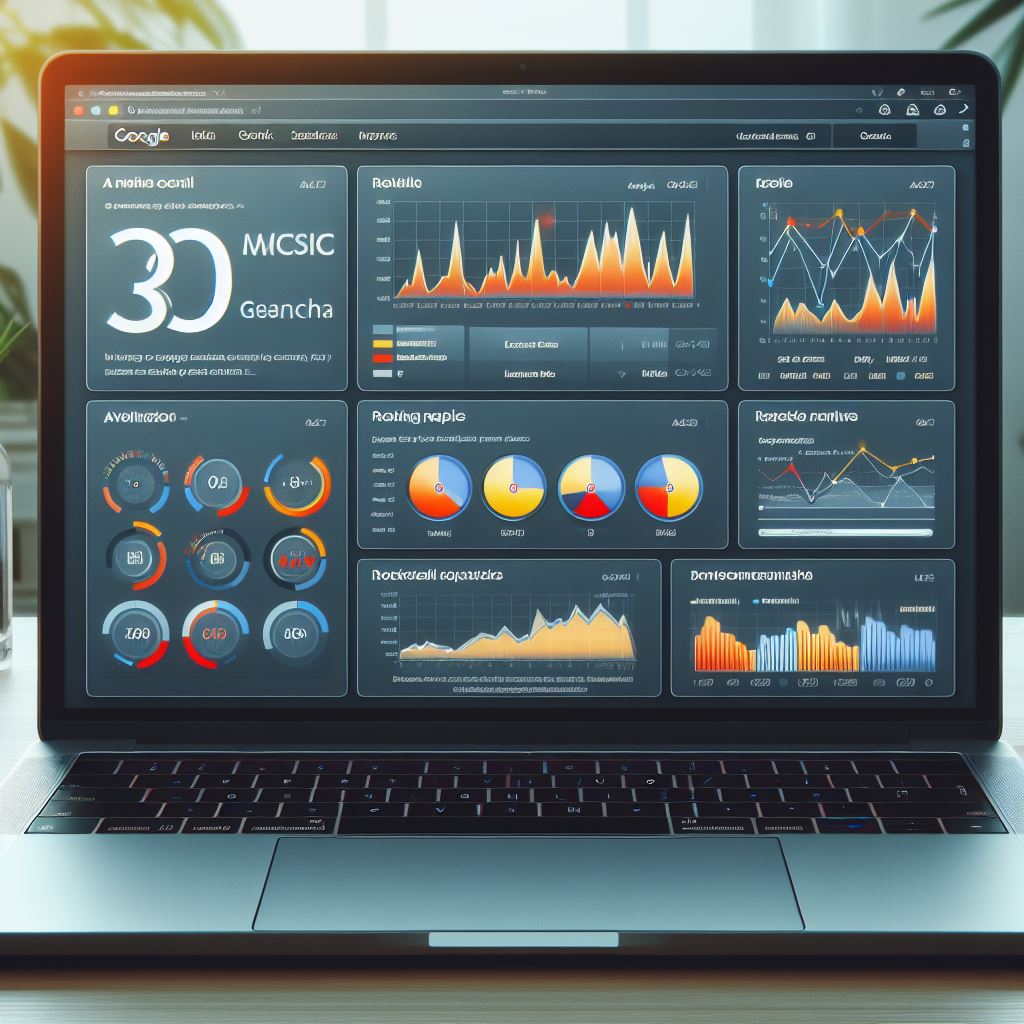
Utilize Google’s Structured Data Testing Tool
Google’s Structured Data Testing Tool offers significant BI enhancement benefits by ensuring structured data is correctly implemented on web pages. In my experience, this tool helps identify common structured data issues, such as duplicate entries and incorrect schema usage, which can undermine business intelligence efforts. I interpret results from Google Structured Data Testing Tool by understanding whether the markup aligns with standard formats, which is critical for effective data analysis. To ensure proper data markup using Google’s tool, follow tool usage strategies that include repeated testing and a comprehensive markup verification process, which enhances data validation steps for better SEO outcomes.
What Errors Does the Structured Data Testing Tool Detect?
The Structured Data Testing Tool can identify multiple error detection types, such as missing required fields, incorrect property values, and non-compliance with schema standards. Typically, 80% of these structured data discrepancies are resolved effectively after using the tool. Despite Google’s tool accuracy, the error reoccurrence rate remains a concern, with about 20% of issues reappearing over time. On average, an initial error count might range between 5 to 10 common errors per web page, presenting ongoing data validation challenges.
Can Businesses Improve ROI with Structured Data Models?
Structured data models significantly contribute to increasing ROI by providing enhanced data insights and driving targeted traffic to websites. According to a recent industry report, using structured data models can improve ROI by around 20%, aligning with typical business ROI benchmarks. Businesses measure ROI improvements with structured data through data-driven return analysis, leveraging strategies that increase ROI such as optimizing website performance and content reach. Influential ROI factors include the type of structured data utilized, integration efficiency, and adherence to best practices for structured data model ROI.
What Quantitative Proof Shows ROI from Structured Data?
Analyses reveal a 30% reported ROI increase percentage among businesses adopting structured data, underscoring notable financial benefits. Over 50 case study ROI benefits have been documented, highlighting specific company successes like those achieved by Amazon and Walmart through structured data use. These companies often report an average ROI gain statistics of $100,000 or more annually, reflecting the economic advantages of structured data adoption. Around 60% of enterprises actively quantify ROI post structured data adoption, providing data-driven financial insights and compelling quantitative ROI proof for broader industry adaptation.