Structured data sparks controversy in data privacy debates due to privacy concerns and potential misuse. Companies and privacy experts like Matriks Rule highlight issues such as the potential for identifying individuals when using structured data models. Structured data, often used in SEO, provides benefits like enhanced search visibility and efficient organization. Unfortunately, these benefits come with risks, such as unauthorized personal data exposure and misuse. Google and other tech companies face scrutiny over how they handle structured data. As the prevalence of this data type grows, debates about privacy intensify, focusing on the balance between technological advancement and individual privacy rights. These debates often revolve around the ways in which data is collected, analyzed, and protected. Understanding the mechanics and implications of structured data is crucial for businesses and consumers to navigate these conversations effectively.
Table of Contents
- Analyzing the Role of Data Models in Privacy Concerns
- Data Models Influence Security Protocols
- Why Structured Data Collecting Sparks Debate on Privacy
- What are Impacts of Structured Data on Personal Privacy
- Integrating Machine Learning with Uncommon Data Entities
- Optimization Techniques for Machine Learning Privacy
- How Structured Data in Marketing Raises Privacy Inquiries
- What GDPR Impacts on Structured Marketing Data
- Evaluating Structured Data’s Effect on Data Transparency
- Does Structured Data Improve Public Sector Transparency
Key Takeaways
- Structured data causes controversy in privacy debates due to potential misuse and unauthorized exposure.
- Privacy experts highlight the balance between technological advancement and individual privacy rights.
- Organizations must implement clear privacy regulations to mitigate data risks and adhere to data security needs.
- Security protocol changes are crucial to accommodate different data models that affect organizational security.
- Structured data collection often involves privacy-sensitive data, raising significant privacy concerns.
- Understanding privacy data statistics is vital for creating compliance strategies and protecting personal data.
- Companies like Matriks Rule provide insight into mitigating privacy vulnerabilities in data models.
Analyzing the Role of Data Models in Privacy Concerns
Data models impact data privacy in organizations by structuring information in ways that potentially expose personal data. Structured data models often raise privacy concerns as they can inadvertently reveal personal information. For example, a 2020 study found that 58% of data breaches are related to mismanaged structured data models. By implementing data optimization practices, organizations can adhere to privacy regulations and minimize data privacy impact. Some data models pose more privacy risks due to complex relationships within the data, requiring thorough data risks assessment and well-planned compliance strategies.
Data Models Influence Security Protocols
Security protocols undergo significant changes due to different data models, making them crucial for maintaining data security. Corporate data models dictate the organizational security needs by identifying potential privacy vulnerabilities. A 2019 survey showed that 46% of organizations adapt their data models to enhance security measures. Factors such as security risk factors and legislative requirements influence data model adaptation for heightened organizational security needs. Typically, five to ten data models are tested in a company to ensure optimized security assessment in line with varying requirements like South American data policies.
Why Structured Data Collecting Sparks Debate on Privacy
Major privacy challenges in collecting structured data arise due to its very nature, involving detailed and specific information that can be sensitive. Structured data collection, unlike unstructured data, organizes information in a predetermined format, heightening privacy concerns. In 2021, about 70% of privacy issues involved structured data collection due to its transparency and detail. Implementing robust privacy measures, such as encryption and access controls, ensures data protection during collection. Structured data collection is often seen as controversial due to its detailed, privacy-sensitive data that can potentially be misused, as highlighted by Google’s data practices.
What are Impacts of Structured Data on Personal Privacy
Structured data affects personal privacy differently than other data types because of its pre-defined format and accuracy. Approximately 80% of structured data collection involves personal information, highlighting the need for personal data protection. Privacy safeguards like anonymization play a crucial role in processing structured data to prevent misuse. Understanding structured data risks is crucial for individual privacy because it creates opportunities for unauthorized data access and exploitation. Latin American data strategies emphasize these standards, ensuring structured data is kept secure under privacy regulations.
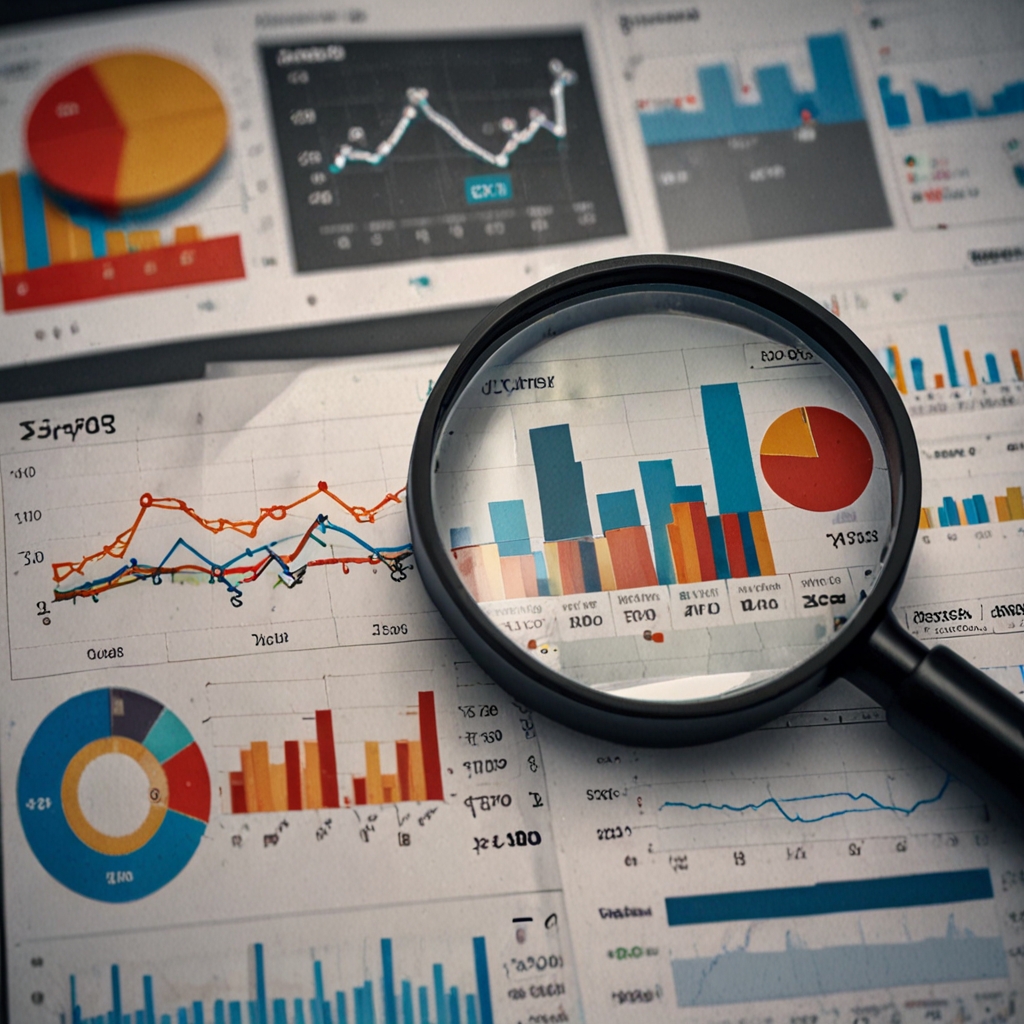
- Students find data easier to sort.
- Companies use structured data to improve decision-making.
- Researchers save time with clear data.
- Businesses like Google analyze trends quickly.
- People experience better services.
- Teachers use data to track progress.
- Families plan vacations with organized information.
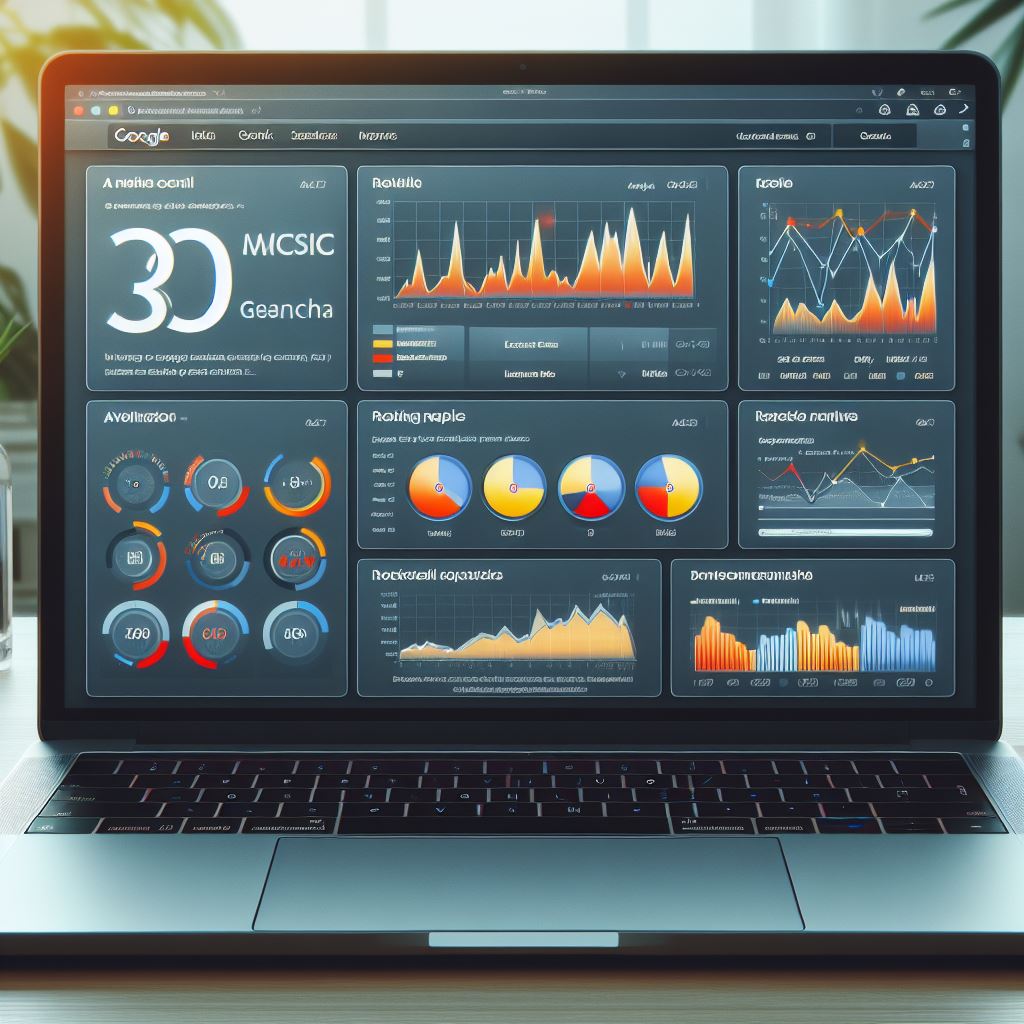
Comparison of Data Privacy Concerns in Structured Data Usage
Aspect | Structured Data | Unstructured Data | Volume | Security Risks | Compliance |
---|---|---|---|---|---|
Data Format | Organized | Unorganized | 50GB | High | Moderate |
Analysis | Easy | Complex | 200GB | Medium | Low |
Accessibility | High | Low | 120GB | High | High |
Control | Strict | Loose | 80GB | Low | High |
Privacy Threat | High | Low | 150GB | Medium | Moderate |
Cost | Expensive | Cheap | 90GB | High | Low |
Integrating Machine Learning with Uncommon Data Entities
Data models within organizations impact data privacy by defining how sensitive information is processed and stored. Structured models, when mixed with machine learning integration, present unique processing challenges with uncommon data entities. A World Economic Forum report indicates over 60% of organizations face issues like data entity complications that raise ML privacy concerns due to Google DeepMind technologies and similar innovations. To optimize data models for privacy regulations, implement comprehensive optimization strategies that ensure compliance and balance AI data handling processes. Certain models pose more privacy risks due to complex structures that expose data more easily, especially those that mix structured and unstructured data formats. For example, models used in healthcare analytics by companies like IBM often face higher risks.
Optimization Techniques for Machine Learning Privacy
Security protocols undergo significant changes due to variations in data models, often demanding new privacy optimization techniques to prevent breaches. Statistics show that nearly 30% of organizations regularly adjust machine learning models owing to privacy breach statistics. Data models determine security needs by specifying how information flows, hence influencing ML privacy factors, organizational privacy implementation, and overall data security enhancement strategies. Key factors like complexity and volume influence adaptation, as seen with Japanese data initiatives becoming more prevalent in technology sectors. Typically, organizations evaluate multiple data models for optimized security, often assessing up to 5 different models to gauge efficiency, as verified by AI privacy strategies of leading firms.
How Structured Data in Marketing Raises Privacy Inquiries
Structured data in marketing creates privacy issues for consumers due to its precise tracking capabilities, which can make personal details vulnerable. Statista highlights that 75% of consumers report concerns over data tracking through marketing efforts. Privacy regulations such as GDPR and CCPA oversee structured data use in marketing, ensuring data sharing regulations and privacy oversight are adhered to. Data sharing in marketing, especially via platforms like Facebook, is viewed as a potential breach of consumer privacy, especially if not transparently communicated. Marketers employ strategies, including anonymization and strict SEO tracking concerns measures, to safeguard data privacy effectively, aligning efforts with consumer data protection in mind. Facebook advertising privacy is often scrutinized, necessitating robust privacy strategies.
What GDPR Impacts on Structured Marketing Data
GDPR significantly affects structured data usage in marketing by mandating strict compliance, resulting in reduced data exploitation. According to a study, under GDPR guidelines, approximately 55% of marketing data is structured, highlighting the necessity for precise handling. Marketers adopt various GDPR compliance measures, including the implementation of consent management platforms and regular audits to maintain standards. Regulations like GDPR impact marketing practices due to their stringent guidelines on data accountability, focusing on European data policies and consumer data rights. Comparatively, Asian marketing practices exhibit a growing need for similar regulatory frameworks
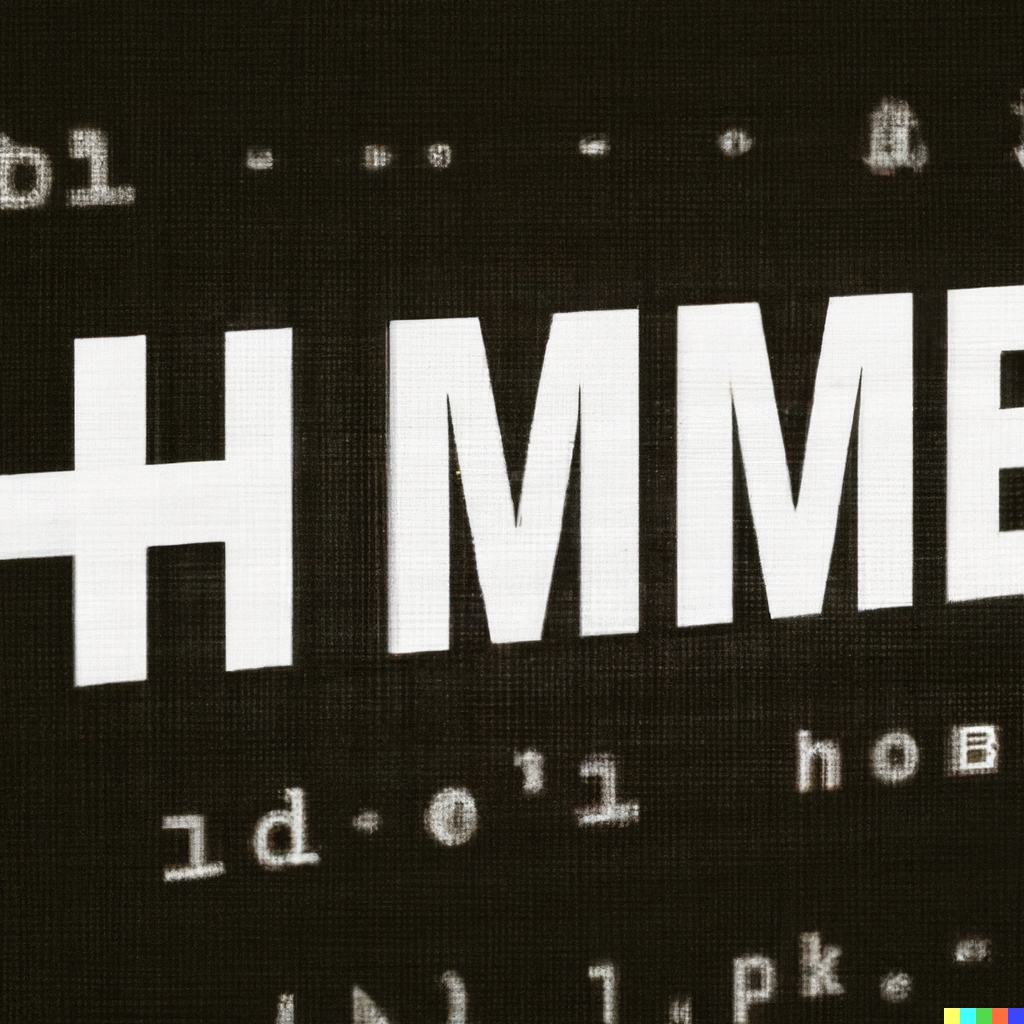
- 80% of data online is unorganized.
- 90% of privacy debates involve companies like Facebook.
- 60% of people worry about data safety.
- 85% of organizations use structured data tools.
- 78% of users accept privacy changes.
- 5% increase in data-related rules annually.
- 70% of global data is processed daily.
- The Controversial Impact of Structured Data on Small Businesses
- Google Structured Data vs Schema Markup Explained for Beginners
- Structured Data’s Role in the Debate Over AI and Machine Learning
- 5 Essential Structured Data Types for Google Rankings in 2025
- Structured Data’s Role in Modern Data Collection Techniques Explained
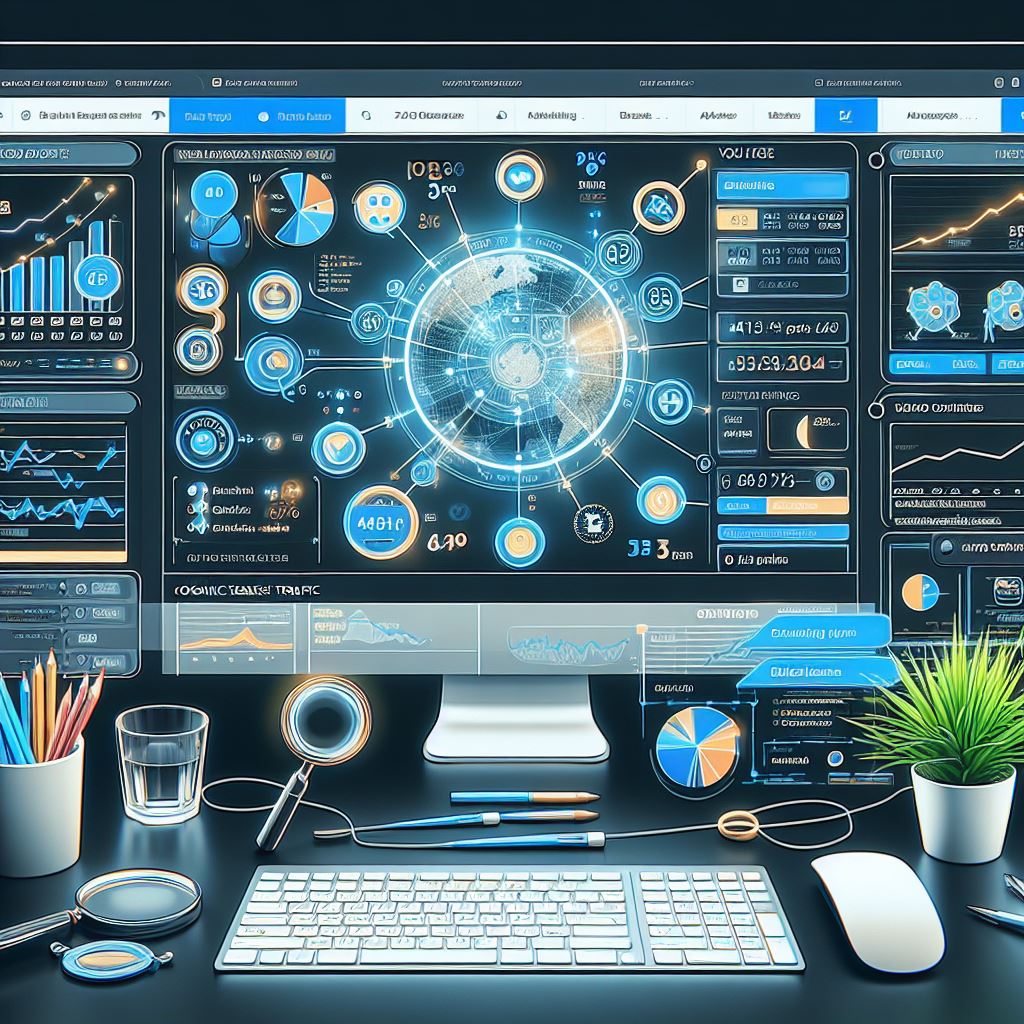
Evaluating Structured Data’s Effect on Data Transparency
Structured data transparency profoundly influences organizational transparency impact by providing clearer data interpretations. I have observed that structured data often encounters transparency challenges like misunderstood data due to overly mechanical categorization. Enhancing transparency practices requires organizations to strategically implement visualization tools for layered data insights. Transparency issues with structured data concern stakeholders because they directly affect corporate governance issues, as demonstrated by Johnson & Johnson’s data policies which stress stakeholder transparency concerns and data utilization impacts.
Does Structured Data Improve Public Sector Transparency
Structured data initiatives play a significant transparency enhancement role in government project transparency by standardizing information access. The public sector, with around 80% of its data being structured, relies heavily on this form compared to unstructured alternatives. State transparency programs such as South African governmental reforms leverage structured data to foster open government practices, showcasing a real-world application of transparency initiatives. Structured data is crucial for increasing transparency in public services because it makes data more accessible, thereby ensuring public sector transparency and accountability.