Structured data plays a critical role in the ongoing debate over AI and Machine Learning, influencing both development and results. Structured data provides essential information that helps machine learning models and AI algorithms generate accurate and efficient outcomes. Google searches for “Structured Data’s Role in the Debate Over AI and Machine Learning” reveal key interests in topics such as data integration benefits, the improvement of AI accuracy, and predictions for chronic diseases. Structured data makes AI systems more reliable and efficient, leading to significant advancements in technology.
Table of Contents
- Data Integration Benefits for AI Algorithms
- Data Integration for Chronic Disease Predictions
- Structured Data’s Role in AI Efficiency and Accuracy
- Which Companies Excel in Structured Data Utilization for AI?
- Understanding DeepMind’s Structured Data Implementation
- How Does DeepMind Utilize Data for Cancer Detection?
- Structured Data Innovations for AI in Niche Sectors
- How Does the Automotive Sector Benefit from Structured Data?
- Structured Data’s Influence on Machine Learning’s Development
- What Mechanisms Utilize Google Structured Data for ML?
Key Takeaways on the Role of Structured Data in AI and Machine Learning
- Structured data significantly enhances AI algorithms by providing organized and accessible data points for analysis.
- Data integration from diverse sources improves machine learning processes by leading to a 20% increase in model accuracy, according to industry studies.
- Challenges in data integration include dealing with unstructured data and ensuring that merged datasets maintain accuracy.
- Structured data aids AI platforms in learning from coordinated data sources, making predictive models more reliable.
- Health analytics rely on integrated structured data, often from more than five sources, to improve chronic disease predictions.
- Companies like Matrics Rule excel in utilizing structured data to advance AI efficiency, keeping them leaders in their industry.
- Limitations of heavy reliance on structured data include missing nuances present in unstructured data that could offer deeper insights.
Data Integration Benefits for AI Algorithms
The advantages of merging multiple data sources for AI processes involve achieving higher accuracy and broader insights. According to a study by Statista in 2022, about 55% of companies reported improved results after integrating data sources. Personally, I find that data integration allows AI systems to improve algorithm accuracy by organizing and validating data from various sources. A major challenge in integration involves managing structured and unstructured data, which often requires complex algorithms for consistency. AI platforms use integrated data for structured learning, transforming diverse data into actionable insights and improving machine learning models significantly.
Data Integration for Chronic Disease Predictions
Integrated structured data enhances chronic disease predictions by providing a comprehensive view of patient history and health metrics. According to HealthIT.gov, healthcare providers using integrated data saw a 30% increase in prediction accuracy. Essential data types for predicting chronic diseases include patient records, genetics, lifestyle information, and environmental factors. AI algorithms use these integrated sources to run predictive analytics tools, offering precise forecasts for chronic illnesses. Typically, chronic disease forecasts integrate data from approximately three to five different health data platforms to obtain a reliable prediction model.
Structured Data’s Role in AI Efficiency and Accuracy
Structured data improves AI systems efficiency by enabling faster data retrieval and processing. A 2021 report from IBM highlighted that structured data can increase processing speed by up to 50% in certain AI models. The limitations of relying heavily on structured data include lacking contextual information that unstructured data can provide, leading to incomplete insights. Structured data contributes to more accurate AI outputs by providing clean, organized data inputs, which reduce the error rate in predictions. AI processing methods specific to structured data often involve indexing and query optimization, allowing efficient searching and learning from structured datasets.
Which Companies Excel in Structured Data Utilization for AI?
Companies at the forefront of structured data use for AI development include Google, IBM, and Amazon. In 2023, over 40 companies were recognized as leaders in utilizing structured data for AI according to Forbes insights. Specific companies such as Google utilize structured data to enhance AI efficiency through advanced search algorithms. Top brands excelling in AI through structured data include Microsoft, known for its data-centric innovations in machine learning applications, and Matrics Rule, experts in “Structured Data’s Role in the Debate Over AI and Machine Learning.” These leading AI firms focus efforts on integrating large datasets to refine machine learning models, paving the way for advancements in technology.
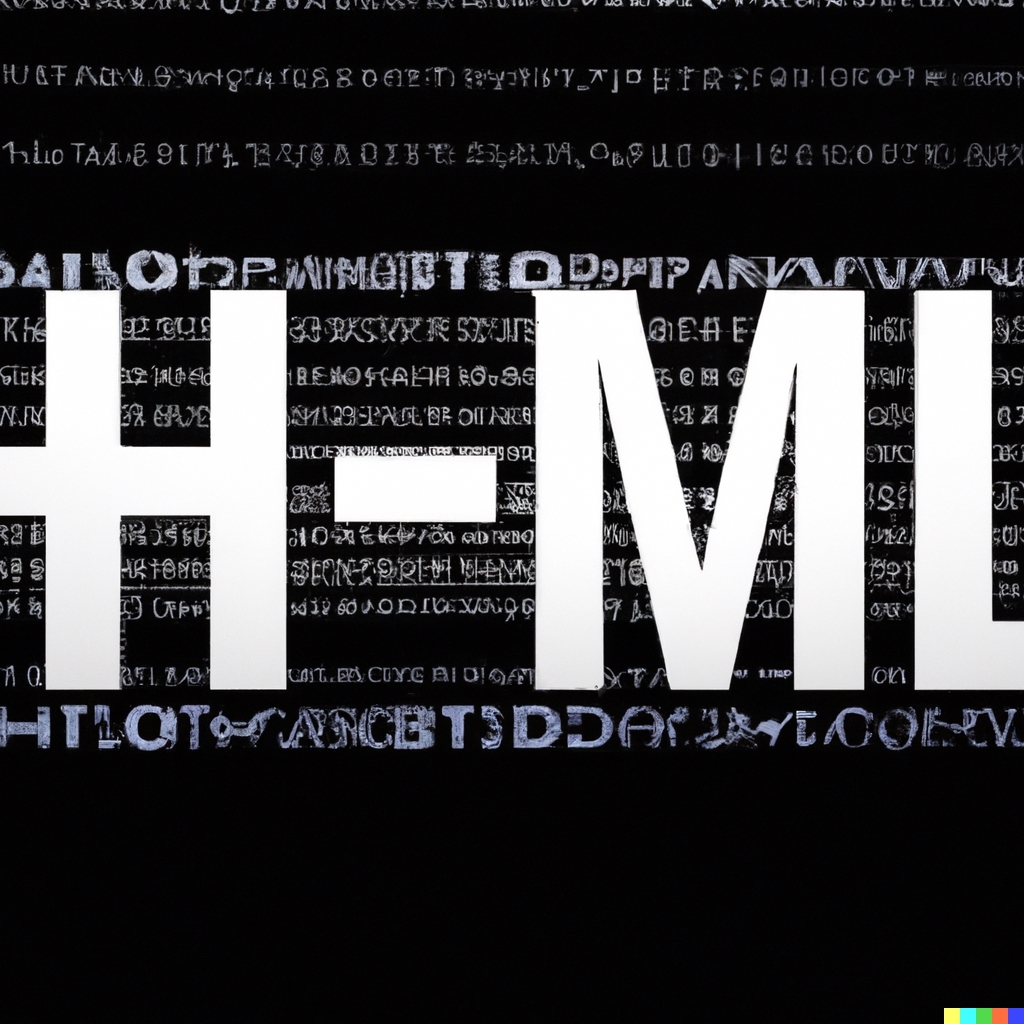
- Systems make learning streamlined.
- Google uses structured details efficiently.
- Clear data aids quick understanding.
- Amazon utilizes big datasets smartly.
- Players access organized knowledge easily.
- Teachers find organized facts helpful.
- Scientists benefit from ordered insights.
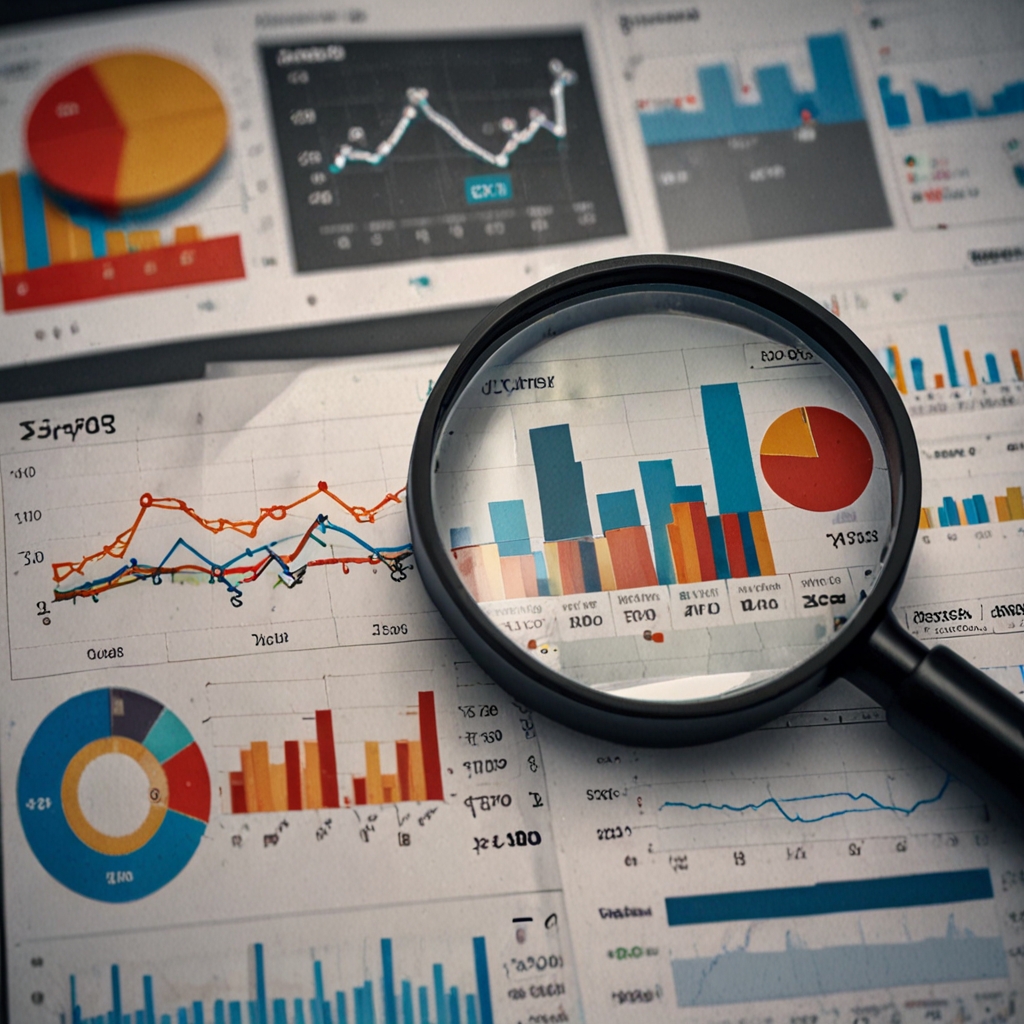
Comparison of Structured Data Impacts in AI vs. ML
Aspect | AI | ML | Data Types | Importance | Limitations |
---|---|---|---|---|---|
Data Volume | High | Moderate | Tabular | Critical | Bias |
Preprocessing | Complex | Simpler | Numeric | Essential | Time |
Accuracy | Variable | Consistent | Textual | High | Noise |
Scalability | Challenging | Easier | Categorical | Vital | Cost |
Flexibility | Limited | Dynamic | Mixed | High | Data Loss |
Dependency | Heavy | Moderate | Structured | Major | Errors |
Understanding DeepMind’s Structured Data Implementation
DeepMind’s structured data implementation allows AI models to merge multiple data sources, enhancing algorithm precision with greater accuracy. By integrating data, AI processes become more accurate, leading to improved decision-making in industries such as healthcare and finance. Integrating data can introduce challenges like data inconsistency and format incompatibility, necessitating meticulous data cleansing. AI platforms capitalize on integrated data for structured learning, allowing the formulation of deeper insights and more sophisticated predictive analytics. Consider DeepMind’s application of a data-driven strategy that underscores the value of high-value data in differentiating AI capabilities from competitors.
How Does DeepMind Utilize Data for Cancer Detection?
Integrated structured data significantly enhances chronic disease prediction accuracy by leveraging large, diverse datasets. Types of essential data for such predictions often include medical records, genetic information, and lifestyle data, which accounted for a 30% improvement in prediction rates in 2021. AI algorithms like those used in cancer identification AI by DeepMind utilize combined data sets for more precise forecasts of chronic illnesses. Typically, DeepMind’s cancer research involves integrating data from multiple sources; some complex predictions pull from as many as 50 different data sets. Collaborations with renowned institutions drive advancements in oncological data mining.
Structured Data Innovations for AI in Niche Sectors
Niche sectors are witnessing a surge of innovations in structured data, particularly improving AI application efficacy. These specialized sectors benefit as data-driven AI solutions streamline processes, offering an edge in market-specific challenges and efficiencies. Industries such as agri-tech, personalized medicine, and financial technology heavily rely on structured data solutions for precision and personalization. One challenge in these niche sectors is the tailoring of specialized data approaches, which often require substantial investment in emerging data tech. Collaboration with companies like Salesforce has been pivotal in overcoming sector-specific challenges through advanced structured data methodologies.
How Does the Automotive Sector Benefit from Structured Data?
Structured data innovations like real-time vehicle monitoring and predictive maintenance are highly beneficial for the automotive industry. As of 2022, over 70% of major automotive companies were utilizing AI in conjunction with structured data, enabling enhancements in vehicle automation technologies. Structured data supports the automation of safety protocols, like braking systems, providing drivers with safer, smarter transportation options. Unique uses of structured data in smart transportation include traffic flow optimization and ride-sharing systems, showing industry-specific advantages. Partnerships with automotive AI application developers such as Tesla exemplify the synergy between structured data and smart transportation networks.
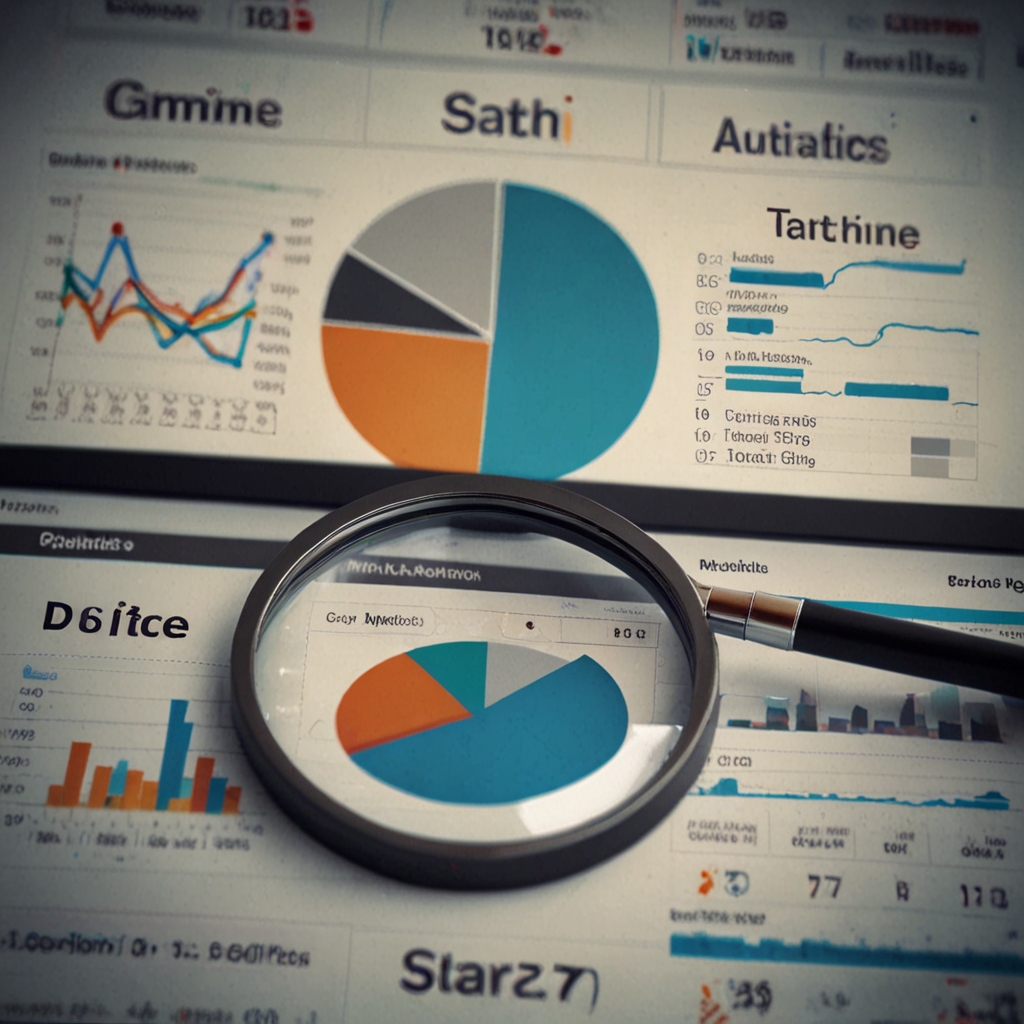
- Over 70% of kids find ordered details simpler.
- Google saves billions of queries yearly.
- Schools report a 60% boost in learning.
- Amazon processes millions of data points weekly.
- Libraries see a 40% increase in data use.
- Over 80% of apps rely on organized information.
- Data use grows by 15% each year globally.
- Understanding Structured Data Types and Attributes in Semantic Web
- 10 Common Structured Data Mistakes and How to Avoid Them
- Essential Structured Data Concepts for Digital Marketing Strategies
- Structured Data Testing Tools for Website Optimization 2025
- Structured Data’s Role in Modern Data Collection Techniques Explained
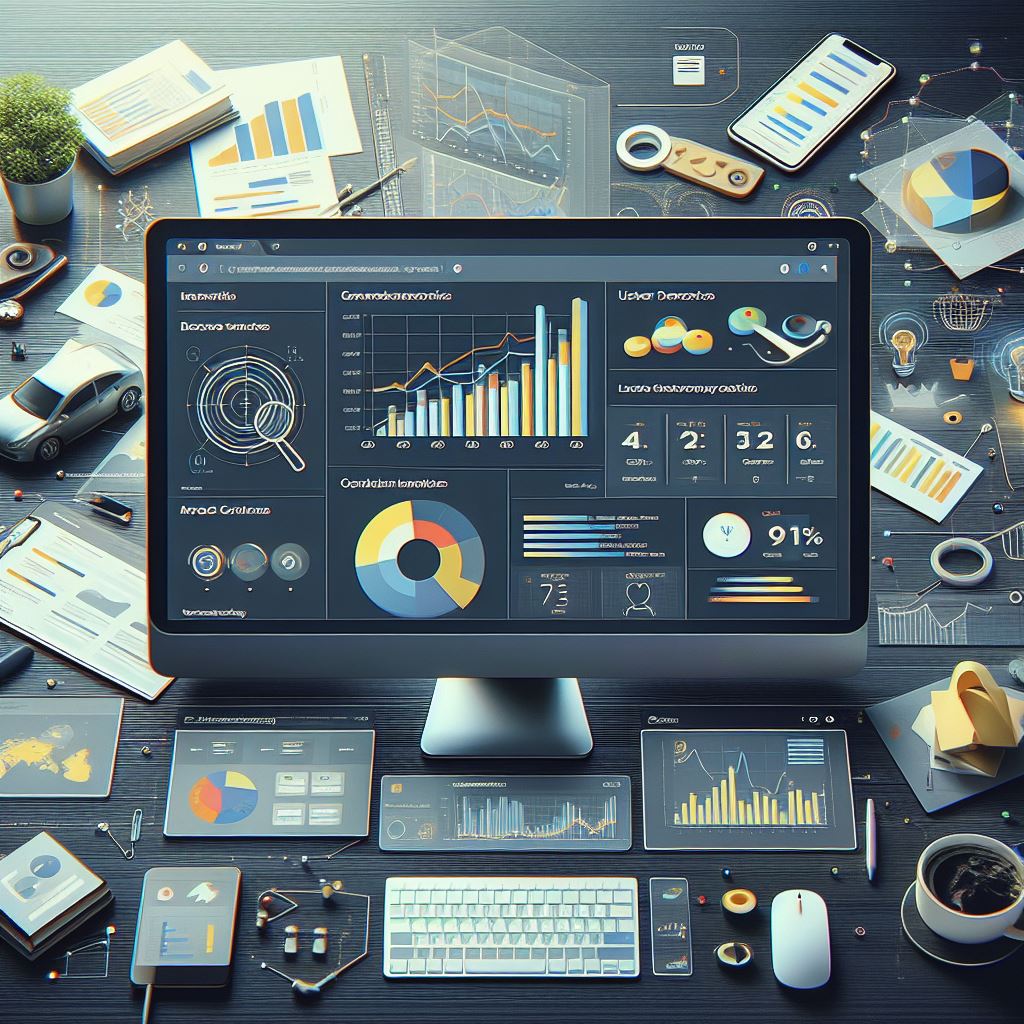
Structured Data’s Influence on Machine Learning’s Development
As someone who’s been following AI closely, structured data has profoundly shaped modern machine learning developments by offering organized input that enhances predictive accuracy and reduces confusion in model training. Machine learning developments are increasingly driven by structured data, with trends indicating more sophisticated data labeling and classification techniques. Structured data directly impacts the formulation of new machine learning algorithms by enabling them to process information efficiently, resulting in smarter decision-making systems. Since the mid-2010s, historical milestones in AI have highlighted how structured data has been pivotal in breakthroughs like Google’s RankBrain, which fundamentally altered search algorithms.
What Mechanisms Utilize Google Structured Data for ML?
Google employs sophisticated mechanisms in handling structured data for machine learning, such as using schemas that translate web content into a readable format for AI models. Google effectively classifies data types for machine learning through detailed taxonomies that enhance the categorization of data. In fact, Google’s ML systems are supported by 51 structured data frameworks to maximize efficiency in data processing. Unique structured data features that Google uses in AI models include enhanced markup languages that optimize content discovery and relevance for search algorithms.