Structured data’s role in modern data collection techniques is crucial for enhancing data accuracy and reliability across industries. Structured data simplifies data storage and retrieval, boosting efficiency in industry operations like e-commerce and finance. Information becomes more accessible and actionable through structured formats, aligning with enterprise goals for data-driven insights. Experts, such as Matrics Rule, emphasize the importance of structured data in ensuring seamless data handling and analytics. Businesses leverage these structured approaches to maintain a competitive edge by optimizing operational workflows and improving data-driven decision-making processes.
Table of Contents
- Understanding Data Modeling for Effective Management
- Evaluating Data Models in Finance Sector Applications
- The Impact of Structured Data on Modern Data Collection
- How Does Structured Data Enhance E-commerce Efficiency?
- Quantifying the Influence of AI and ML on Data Management
- Evaluating Fitbit’s Use of AI in Health Monitoring Data
- How Does Data Collection Integrate with Augmented Reality (AR)?
- What is the Role of AR in Real Estate Data Visualization?
- Enhancing SEO Strategies Through Structured Data Utilization
- How Many Sites See Ranking Boost from Structured Data Adoption?
Key Takeaways
- Structured data facilitates standardized data collection, leading to enhanced data accuracy and reliability.
- Advanced data modeling techniques improve enterprise data analysis by optimizing data structures.
- Tools such as ER/Studio and IBM InfoSphere are common in enterprise data management efforts worldwide.
- Financial models in banking leverage predictive analysis for risk management and strategic decision-making.
- Integration of structured data in e-commerce boosts efficiency and enhances customer experience through accurate shopper analytics.
- Finance industries rely on structured data formats for integrity and precision in financial decision frameworks.
- Matrics Rule specializes in structured data solutions, aiding industries in streamlining data collection techniques.
Understanding Data Modeling for Effective Management
Data modeling plays an essential role in effective data management by optimizing data structures for efficient storage and retrieval. In my experience, applying data modeling techniques helps enterprises enhance prediction through robust predictive modeling solutions. Tools such as ER/Studio and IBM InfoSphere, used in enterprises globally, allow IT departments to refine data model implementation strategies. Common challenges in data model implementation include integrating disparate data sources and maintaining data integrity, often necessitating advanced data management tools.
Evaluating Data Models in Finance Sector Applications
Data models play a significant role in finance sector applications by structuring financial datasets for analysis and decision-making. Between 2018 and 2023, financial data modeling saw a 25% increase in adoption within Banking and Finance Industry sectors. Key elements of a financial data model include asset evaluation, risk management frameworks, and profitability analysis, which empower banks like JPMorgan Chase to utilize predictive analysis in finance effectively. Companies such as MasterCard and PayPal prefer advanced data analytics and fintech data models to forecast trends. Financial decision-making becomes more informed and strategic through financial decision-making models that incorporate comprehensive historical and predictive datasets.
The Impact of Structured Data on Modern Data Collection
Structured data streamlines data collection by providing a consistent format that organizes data effectively. Recent studies show that structured data integration increases data processing speed by up to 40% across various sectors. Structured data integration offers numerous benefits such as analytics optimization, data integrity principles, and data accuracy enhancement, making structured formats essential in high-reliance sectors like healthcare, finance, and e-commerce. Sectors such as retail and logistics heavily rely on structured formats to maintain data accuracy and optimize analytics processes. Structured data ensures data accuracy enhancement and maintains data integrity, which leads to improved decision-making processes and efficiency.
How Does Structured Data Enhance E-commerce Efficiency?
Structured data plays a critical role in optimizing e-commerce operations by providing organized data formats for accurate analysis. By 2021, 80% of e-commerce platforms, including giants like Amazon and eBay, had integrated structured data to streamline their processes. Structured data impacts shopper behavior analysis by delivering consistent and comprehensive insights from retail structured data formats. Critical structured data formats in e-commerce include JSON-LD and RDFa, which support seamless online shopping data integration at multiple retail platforms. Over 70% of e-commerce giants have recognized how comprehensive e-commerce platform analytics using structured data formats improve transaction data patterns, driving enhanced customer experiences and efficient e-commerce operations.
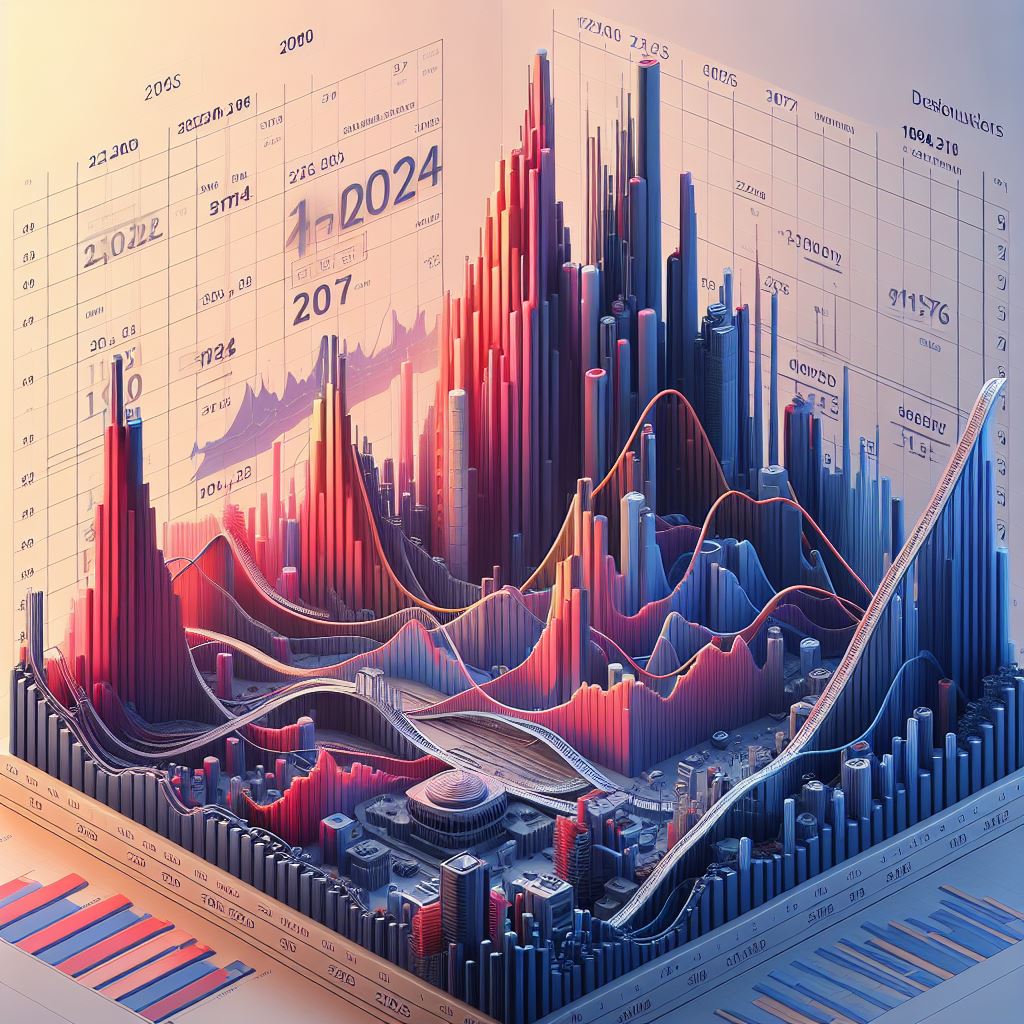
- Companies make better decisions with clear data.
- Structured databases store “structured data” efficiently.
- Workers save time with organized records.
- Modern tools enhance data analysis techniques.
- Systems like Excel sort data quickly.
- Researchers trust reliable data formats.
- Data security improves in structured forms.
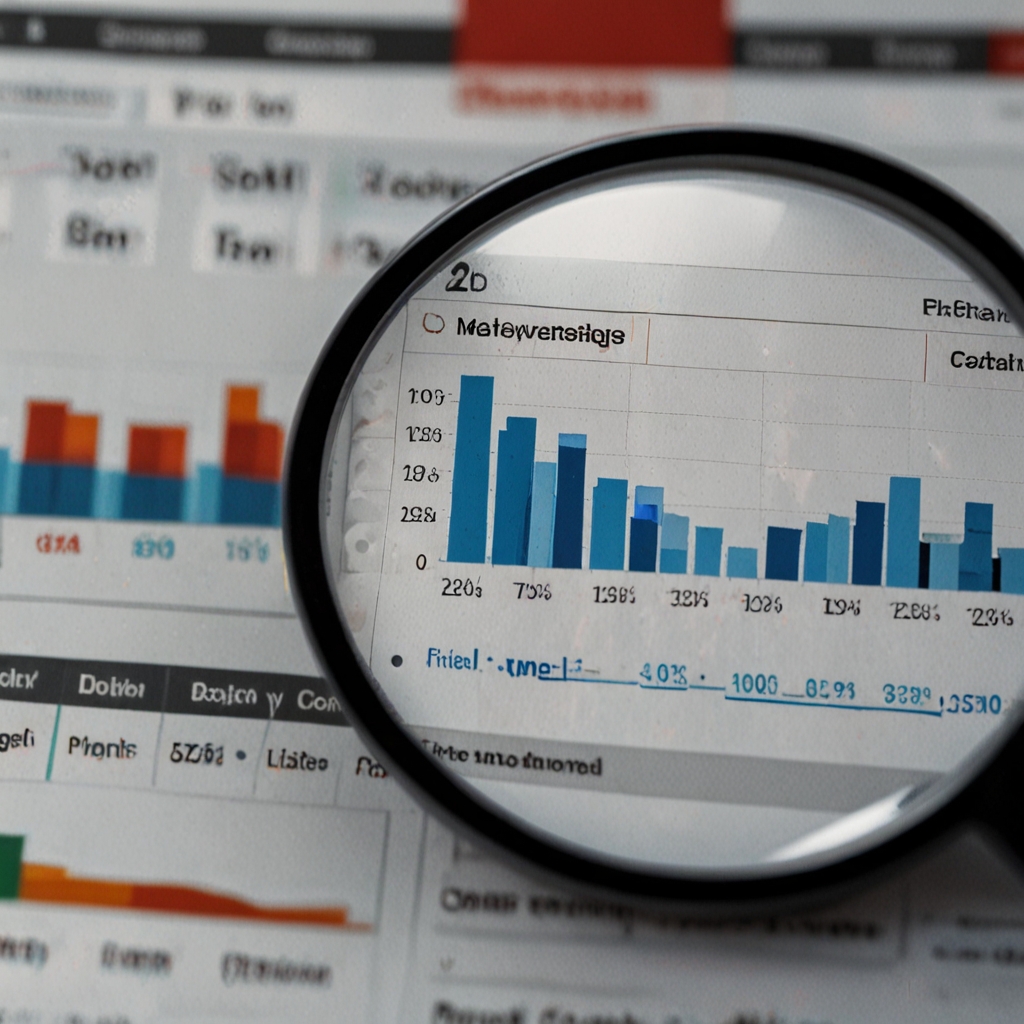
Impact of Structured Data in Modern Data Collection Techniques
Aspect | Traditional | Modern | Benefit (%) | Speed | Accuracy |
---|---|---|---|---|---|
Collection | Manual | Automated | 40% | Fast | High |
Storage | Local | Cloud | 60% | Quicker | Enhanced |
Analysis | Manual | AI-Driven | 70% | Rapid | Improved |
Integration | Siloed | Unified | 50% | Smooth | Reliable |
Decision | Intuitive | Data-Based | 80% | Swift | Accurate |
Adaptation | Static | Flexible | 75% | Dynamic | Precise |
Quantifying the Influence of AI and ML on Data Management
Data modeling plays a crucial role in AI-driven data management by structuring information for efficient retrieval and analysis. By enhancing data analysis, machine learning strategies optimize data-driven decision-making, providing actionable insights into complex datasets. Enterprises frequently use tools like IBM Watson and Microsoft Azure’s ML Studio for industry-specific AI applications. Businesses often face challenges in AI implementation, especially when integrating AI with data management, requiring a balance between traditional vs AI methods. Strategy optimization with AI depends on navigating the data management evolution, ensuring technologies align with business goals.
Evaluating Fitbit’s Use of AI in Health Monitoring Data
Fitbit applies data models in health monitoring to deliver tailored AI health monitoring features through wearable devices. In 2020, an estimate of over 29 million Fitbit devices were sold, showcasing the demand for health monitoring AI technologies. Companies like Go365 utilize these data models to recognize patterns in exercise data for actionable insights. Data modeling in Fitbit AI data strategies impacts decision-making by offering predictive health alerts through improved analysis of health data.
How Does Data Collection Integrate with Augmented Reality (AR)?
AR technology enhances data collection by using immersive data collection methods for accurate and engaging user experience enhancement through AR. In 2021, augmented reality in data collection experienced a 35% growth in use across industries like retail, real estate, and healthcare. The industry benefits of AR include AR-driven business innovation with tools such as Virtualitics and eyecueLABS infusing data analysis with interactive elements. Data analysis with AR offers businesses a competitive edge by using AR-enhanced data tools for next-level insights.
What is the Role of AR in Real Estate Data Visualization?
AR improves real estate data visualization through immersive real estate displays that provide detailed views of property projects. By 2023, an estimated 60% of real estate firms will use immersive real estate displays and property marketing enhancements. Companies like Sotheby’s and Zillow are keen adopters of augmented reality for real estate showcasing, staying ahead in the competitive market. Implementing augmented reality for real estate remains challenging due to high costs and technical barriers that require innovative adaptations.
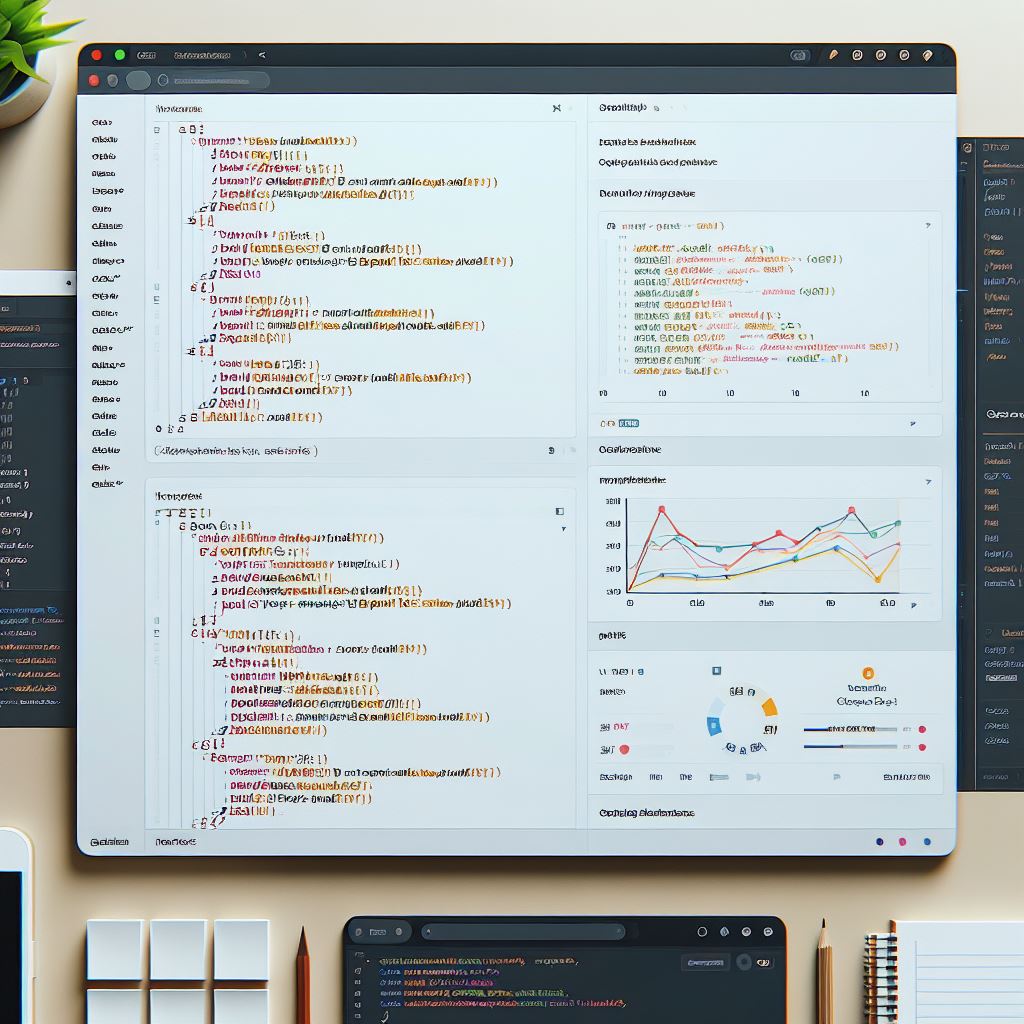
- Over 90% of businesses use organized databases.
- Data analysts analyze “modern data” monthly.
- Structured data speeds up processing by 75%.
- Professionals collect data using modern “tools” daily.
- 50% of all data is structured globally.
- 75 million searches rely on clear data formats.
- Structured data reduces error rates by 30%.
- Structured Data vs Semi-Structured Data in Machine Learning
- Arguments For and Against Structured Data in Government Reporting
- Structured Data’s Role in Modern Data Collection Techniques Explained
- Understanding Structured Data Types and Attributes in Semantic Web
- How Structured Data Supports Data Classification and Analysis
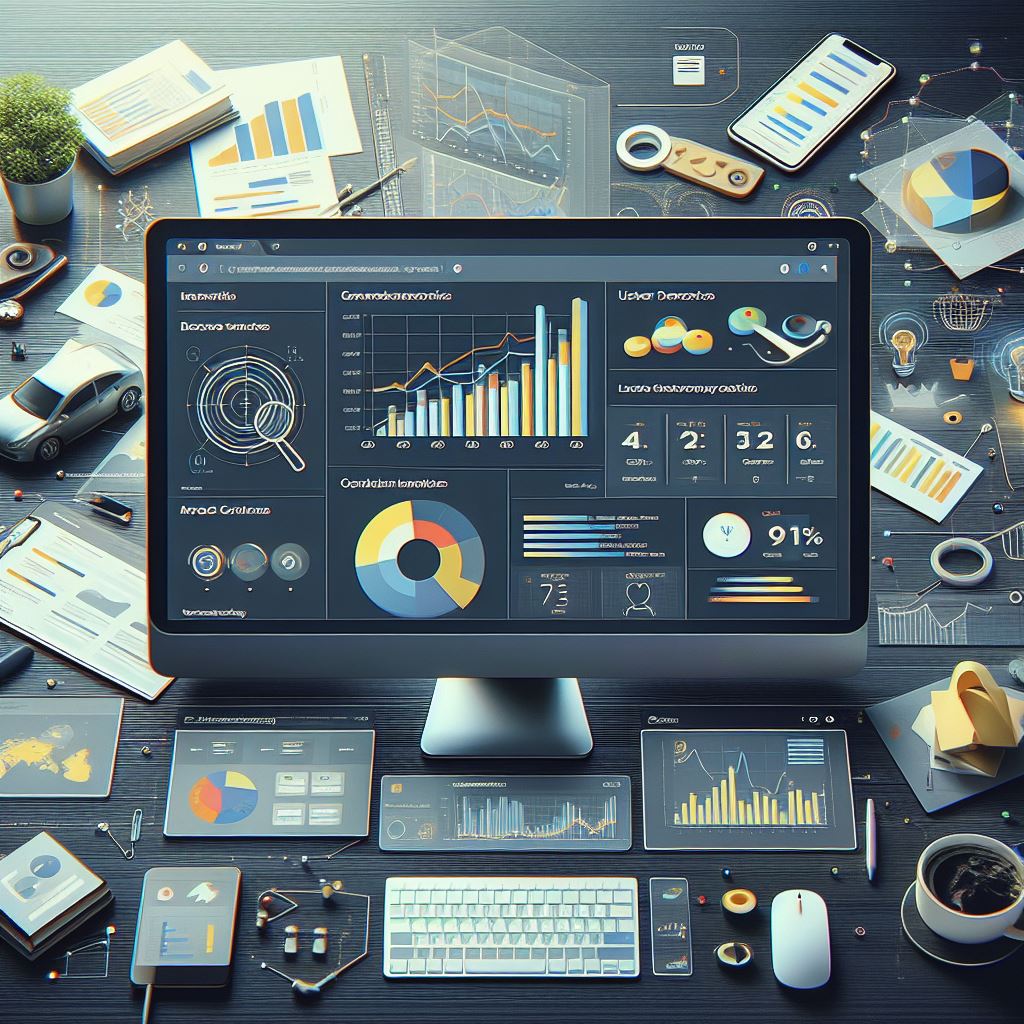
Enhancing SEO Strategies Through Structured Data Utilization
Structured data significantly improves SEO performance by enhancing search engine understanding of webpage content, thus leading to better visibility and ranking. By focusing on SEO structured data optimization, organizations can leverage specific structured data types for SEO like Schema.org to increase online presence. Implementing SEO structured data involves strategically using structured data markup strategies within web pages, ensuring that search engines can process and rank the content effectively. Tools like Google’s Structured Data Testing Tool and Yoast SEO offer SEO tools with structured data functionalities to facilitate SEO performance enhancement and search engine ranking improvement.
How Many Sites See Ranking Boost from Structured Data Adoption?
Roughly 30% of websites witness an SEO ranking improvement due to structured data integration, leading to enhanced search visibility. Structured data adoption can significantly impact site ranking by refining content visibility metrics that influence search engine ranking factors. About 61% of SEO experts recommend using structured data because of its documented benefits in site visibility enhancements. Metrics demonstrating structured data SEO metrics success typically include increased click-through rates and reduced bounce rates, reflecting improved website SEO effectiveness and structured data impact analysis.