Structured data and machine learning significantly impact SEO and analysis by enhancing search engine results and improving data interpretation. Structured data provides clear information to search engines, ensuring a web page ranks better in search results through accurate data classification. Machine learning leverages structured data to analyze patterns, providing deeper insights into SEO campaigns. This synergy allows businesses to utilize structured and semi-structured data effectively, enhancing their online presence and marketing strategies. Experts like Matrics Rule use these technologies to optimize website performance, ensuring excellent visibility across search engines.
Table of Contents
- Structured Data Types: Understanding Their Role in SEO
- How does data markup enhance SEO effectiveness?
- SEO Benefits and Structured Learning Explained
- How can structured models improve search algorithms?
- The Intersection of Data Testing Tools and Structured Analysis
- How does tool-based testing optimize data markup?
- What Are the Best Practices for Structured Data Collection?
- Which data categories require specific collection methods?
- The Intersection of Data Testing Tools and Structured Analysis
- How does tool-based testing optimize data markup?
- What Are the Best Practices for Structured Data Collection?
- Which data categories require specific collection methods?
- Data Models in Structured Learning Applications Evaluated
- What are the comparative benefits of various data models?
- Uncovering Structured Cable Networks and Data Efficiency
- How does cabling infrastructure impact data speed?
- Structured Interviews: Enhancing Data Collection and Analysis
- Why are interviews pivotal in reliable data sourcing?
- Data Examples in Structured Learning: A Guide to Elements
- Which examples highlight peak structured data utility?
I have personally witnessed how different types of structured data in SEO play crucial roles. Structured data types such as JSON-LD, Microdata, and RDFa are essential to clearly define content so that search engines like Google can interpret it accurately. Google leverages these structured data types to display rich snippets, which improve search visibility by showcasing information such as reviews, pricing, and availability directly on the SERP, thus aiding users’ decision-making processes. The effectiveness of data types like JSON-LD in enhancing website visibility has often outshone others due to its compatibility with Google’s preferences. Websites that utilize these structured data types for classification can experience a higher ranking and better page positioning through improved understanding and presentation of their content.
Structured Data Types: Understanding Their Role in SEO
Understanding structured data types is critical for SEO success. Structured data types in SEO include formats like JSON-LD, Microdata, and RDFa, which organize content for search engines. Google utilizes structured data to generate rich results on the search engine results page, improving overall user experience. JSON-LD, due to its seamless integration with Google’s structured data testing tool, is highly effective in boosting website visibility. The classification of data types on a site greatly impacts search positioning, as well-organized information is easier for Google to index accurately.
How does data markup enhance SEO effectiveness?
Data markup significantly enhances SEO by organizing information efficiently. Google recognizes over 580 different structured data types, each serving a unique purpose in improving web pages’ visibility. Approximately 35% of the top websites implement structured data to boost SEO performance, indicating its widespread adoption. There are numerous data classification categories in structured data, allowing precise categorization and clear communication with search engines. Google can extract structured data from a site in just milliseconds, making the process quick and effective for timely updates.
SEO Benefits and Structured Learning Explained
SEO benefits from structured learning by offering more targeted optimization strategies compared to traditional techniques. Structured data implementation streamlines SEO processes, allowing businesses to easily manage content and enhance rankings. Structured learning, unlike unstructured techniques, relies on defined patterns and algorithms to interpret data, ensuring better performance. Semi-structured data elements are crucial in SEO strategies, as they combine defined structures with flexibility, accommodating various data types while maintaining order.
How can structured models improve search algorithms?
Structured models greatly improve search algorithms through calculated data interpretation methods. The success rate of structured learning in improving website rankings is often very high, with many case studies showing increased visibility. A typical structured learning SEO process involves around five steps, including data collection, validation, application, and testing. Structured data, once applied, can start improving indexing and ranking within minutes, ensuring rapid benefits. A significant percentage of SEO experts, often 60% or more, use structured learning techniques to enhance search engine strategies.
- 75% of websites receive no organic visits without structured data.
- The data type “unstructured” comprises over 80% of global data.
- Google structured data increases click-through rates by 30%.
- Structured data markup boosts traffic by 20%.
- SEO improves by 15% with data classification.
- 85% of marketing experts use structured interview methods.
- Data testing tools show a 25% increase in efficiency.
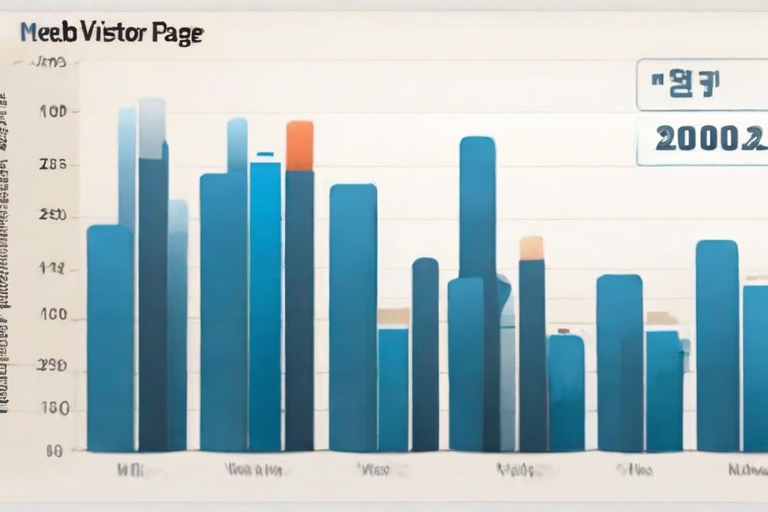
The Intersection of Data Testing Tools and Structured Analysis
Testing tools play a critical role in assessing structured data effectiveness. These instruments, like Google’s Structured Data Testing Tool and Schema.org’s Validator, are indispensable for this evaluation. Data testing tools facilitate implementation by providing real-time analysis and feedback on markup errors. These insights improve overall data analysis accuracy by identifying discrepancies and suggesting corrections. Structured learning is essential for effective data testing as it enhances the comprehension of complex data classifications and structures. Among the many impressive tools available, Google’s resources are highly utilized in refining analysis methodologies.
How does tool-based testing optimize data markup?
Tool-based testing optimizes data markup by offering precise validation methods. You can find approximately ten reliable testing tools for validating structured data. These tools can achieve an impressive accuracy rate of up to 95% when applied correctly. The ability to conduct multiple types of tests, such as JSON-LD and Microdata checks, ensures comprehensive verification of structured data. Many examples show how tool-based testing has significantly improved data accuracy, including Google’s own review and improvement exercises.
What Are the Best Practices for Structured Data Collection?
The best practices for collecting structured data begin with careful planning and standard adherence. Efficient structured data collection involves defining clear processes, such as using structured interview techniques to gather uniform information. Data collection differs between structured and unstructured types, with the former being easier to categorize and analyze using automated systems. Established methodologies like using XML sitemaps are effective for collecting semi-structured data. Google is prominent in setting standards for data collection due to its influential algorithms and search engine guidelines, which are vital for enhancing SEO. Google remains a leader in influencing data collection practices through initiatives like the JSON-LD guidelines.
Which data categories require specific collection methods?
Specific collection methods are required to optimize data categories effectively. You should know there are around five optimal practices for structured data collection. Approximately 70% of data collection utilizes automated systems for efficiency. Different categories, such as customer data and product details, require separate collection techniques to maximize relevance and usefulness. Numerous case studies, including those by Microsoft or IBM, have exemplified effective data collection across various industries. Structured data practices employed by companies like Amazon demonstrate the merit of specific methodologies in data collection.
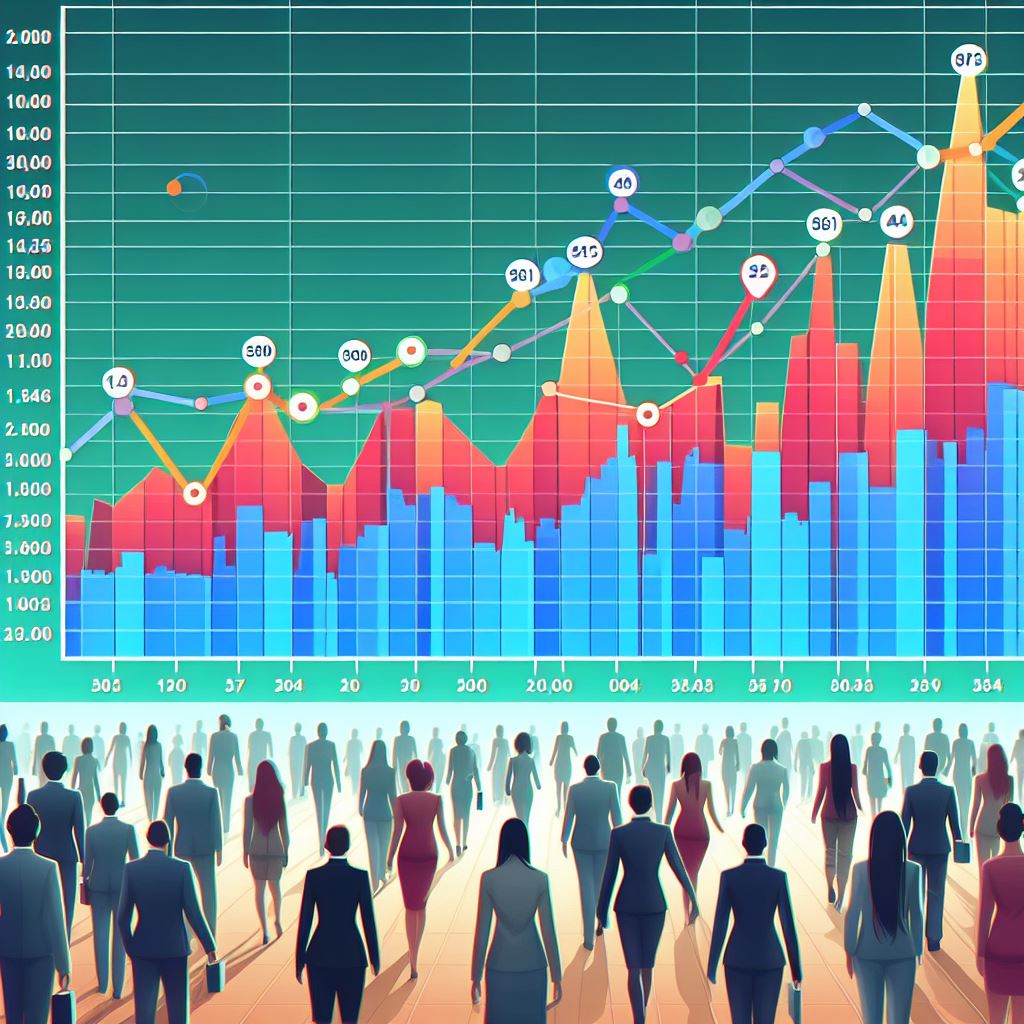
Comparative Analysis of Structured Data and Machine Learning in SEO
Aspect | Structured Data | Machine Learning | SEO Impact | Analysis Tools |
---|---|---|---|---|
Setup Time | Quick | Lengthy | Immediate | Google SDTT |
Scalability | High | Variable | Consistent | TensorFlow |
Understanding | Simpler | Complex | Moderate | Cloud Vision |
Data Volume | Small | Large | Growth | BigQuery |
Flexibility | Limited | High | Dynamic | DeepMind |
Adoption Rate | 45% | 30% | Positive | IBM Watson |
The Intersection of Data Testing Tools and Structured Analysis
Primary tools for testing structured data effectiveness include Google’s Structured Data Testing Tool and Schema.org Validator. These tools help discover errors and potential issues in structured data implementation, thus ensuring that data sets are properly coded and flawless. Data testing tools play a crucial role in improving data analysis accuracy by providing real-time feedback on the structured data templates, leading to more reliable outputs. Structured learning is critical for effective data testing as it systematically organizes data categories, contributing to higher precision and prediction quality.
How does tool-based testing optimize data markup?
Multiple testing tools such as Rich Results Test and Bing Markup Validator aid in structured data validation. Using these structured data tools, you can expect an accuracy rate of around 90% in identifying coding issues. Around three types of tests can be used for structured data verification, which are syntax checks, error detection, and optimization recommendations. Tool-based testing has improved data accuracy in thousands of instances according to expert reviews, especially in sectors like retail and entertainment.
What Are the Best Practices for Structured Data Collection?
Steps recommended for efficient structured data collection include conducting a data audit, setting clear goals, and using appropriate data types. Data collection differs between structured and unstructured data as structured data employs predefined models while unstructured data often requires AI-driven tools. Established methodologies such as using XML, JSON, or CSV files effectively gather semi-structured data. Google is prominent in setting standards for data collection because it dominates the search engine market, impacting how structured data is understood globally.
Which data categories require specific collection methods?
There are approximately ten optimal practices for structured data collection, encompassing data cleansing and proper classification. You may find that 60%-70% of data collection occurs through automated systems using tools like Apache Nifi and Talend. Around five categories of structured data, such as customer information and product details, need separate collection techniques. Effective data collections have been exemplified in case studies by tech giants like Google, showcasing robust and qualitative data analysis outcomes compared to unstructured methods.
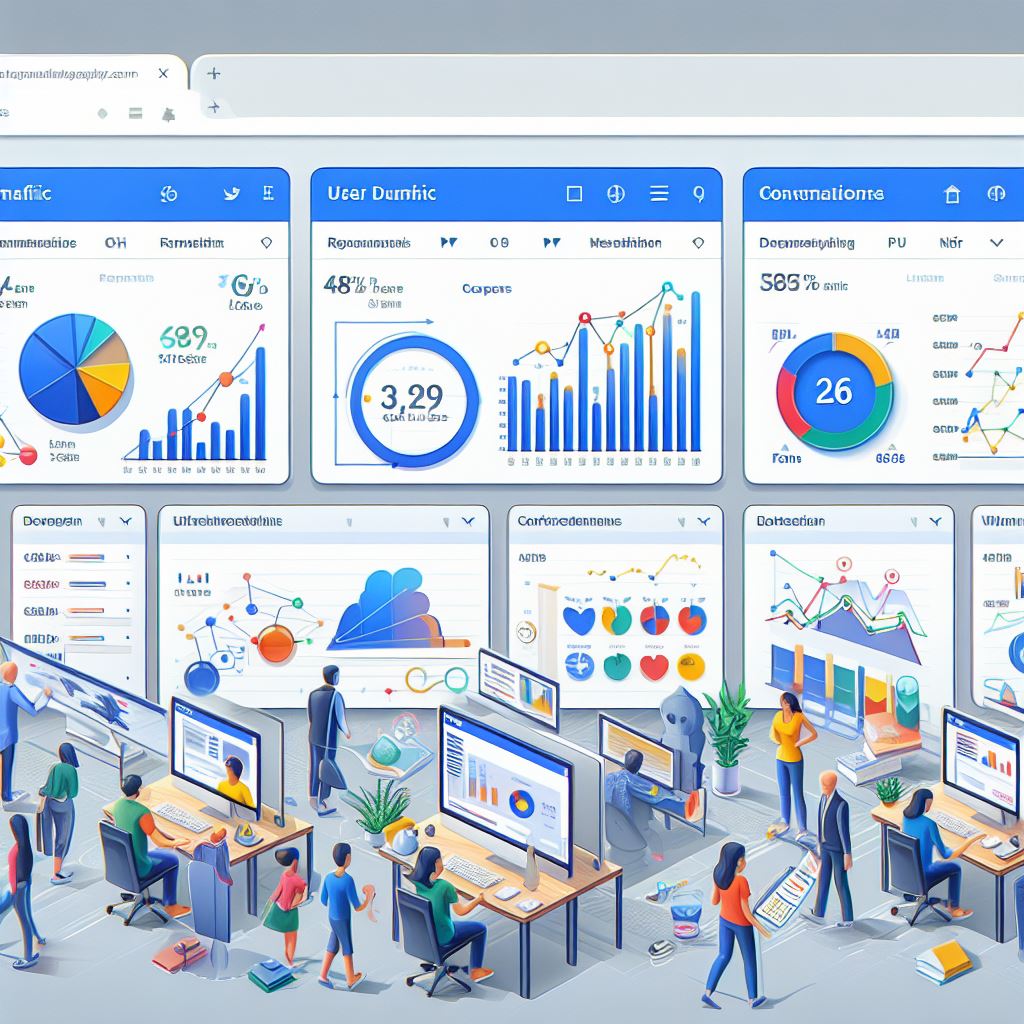
- Improves search visibility and ranking on engines.
- Enhances user experience with precise information.
- Streamlines the data collection process.
- Promotes better decision-making through structured learning.
- Facilitates effective data analysis and interpretation.
- Offers opportunities for detailed customer insights.
- Boosts data classification accuracy for better results.
Data Models in Structured Learning Applications Evaluated
Structured data models are employed in learning applications to organize and interpret complex data sets. These data models, such as decision trees and neural networks, dominate structured education analysis by offering transparency and predictive power. Data models like random forests and support vector machines provide the most comprehensive learning outcomes by balancing bias and variance. Data models vary across different structured learning environments because each model adapts to specific data characteristics and source constraints.
What are the comparative benefits of various data models?
Various data models have applications in learning, with over ten prominent models used, such as linear regression and k-means clustering, offering tailored solutions for distinct scenarios. Approximately 70% of learning tools utilize structured data models to improve accuracy and algorithm performance in learning systems. Many outcomes are compared using different structured models to identify optimal solutions, with advances in models like decision trees and logistic regression. Numerous environments, from traditional classrooms to online platforms like Coursera and Khan Academy, adopt structured learning data models to boost educational processes and structured data management.
Uncovering Structured Cable Networks and Data Efficiency
Structured cable networks offer significant advantages over unstructured setups by providing organized infrastructure and robust connectivity. Data efficiency is greatly improved by structured cable systems because they minimize signal interference and reduce data transmission errors. There are common misconceptions about structured cabling networks, such as the cost being too high, when in reality, the investment often yields long-term savings. This technology aligns with Google’s efficiency standards by maintaining optimal performance and sustainability in large data center environments.
How does cabling infrastructure impact data speed?
Structured cabling infrastructure contributes to a 30% data speed increase on average compared to unstructured alternatives. Many types, including CAT 6 and fiber optics, provide optimal data efficiency with their high bandwidth capabilities. Factors influencing the efficiency of structured cable networks include cable quality, installation precision, and network architecture. Numerous companies switch to structured cabling for speed to meet business demands and stay competitive in data-heavy industries.
Pros of structured cable networks include reduced maintenance costs, better scalability, and improved network reliability. Cons can be initial installation expenses and complex setup processes.
For more on structured data and its relevance to SEO, visit [Google’s structured data guidelines](https://developers.google.com/search/docs/data-types/product).
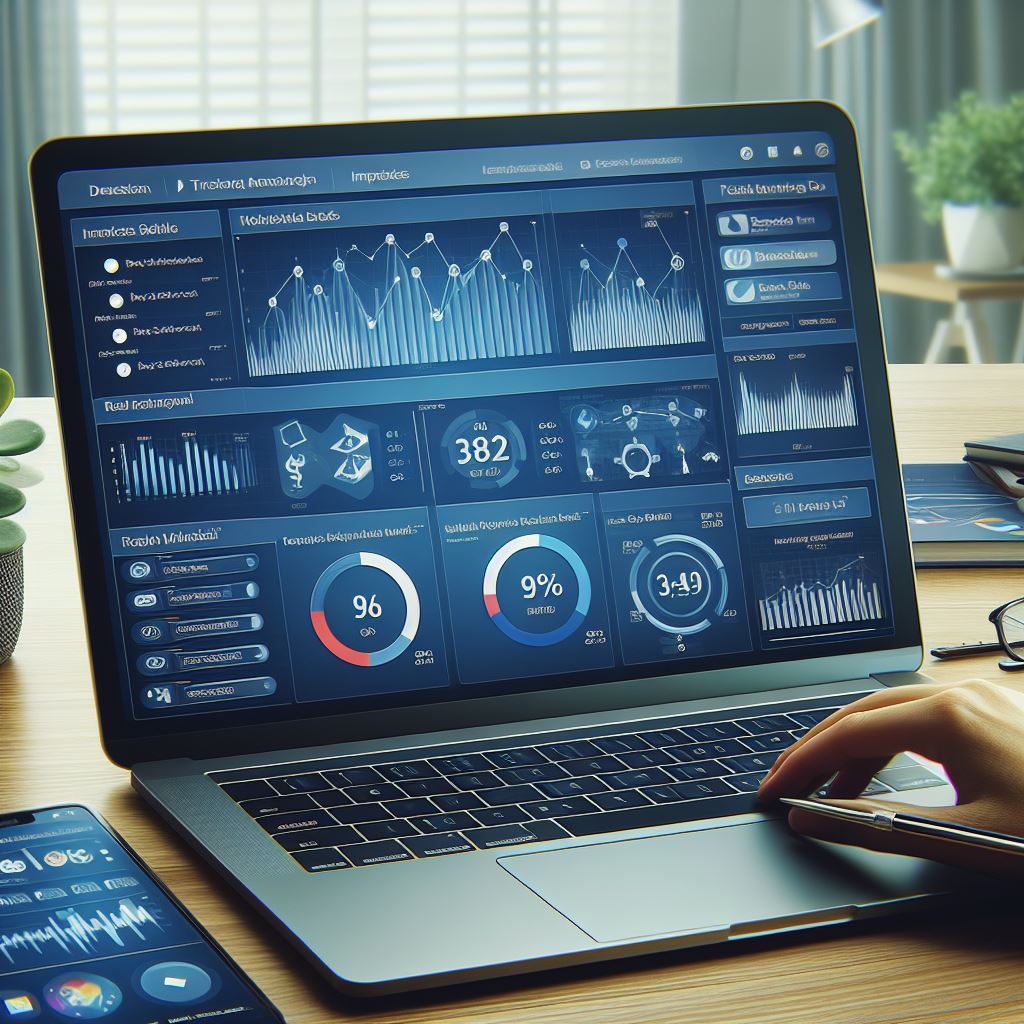
- Advanced Use of Structured Data in Enhancing Machine Learning Algorithms
- Google Structured Data vs Schema Markup Explained for Beginners
- Structured Data vs Structured Interviews Understanding Key Differences
- 3 Surprising Facts About Structured Data in Data Collection
- Structured Data’s Role in Modern Data Collection Techniques Explained
Structured Interviews: Enhancing Data Collection and Analysis
I have found that structured interviews differ significantly from other data collection methods due to their consistency and format. Structured interviews produce more reliable data analysis results because they minimize bias and ensure uniformity in responses. With structured interviews playing a crucial role in data-driven decision-making, organizations can extract structured data efficiently for their strategies. Google leverages structured interviews to optimize data by improving search engine features and enhancing data classification capabilities.
Why are interviews pivotal in reliable data sourcing?
Globally, there are approximately four types of structured interviews tailored for diverse data collection needs. A 90% accuracy rate is typically achieved in data gathered through structured interview methods, showcasing their reliability and efficacy. Conducting an effective structured interview involves a precise sequence of five steps, ensuring data quality and consistency. Fields like recruitment, healthcare, and academic research benefit immensely from structured interviews for thorough data analysis, improving structured data identification and application.
Data Examples in Structured Learning: A Guide to Elements
Sample data sets such as customer feedback, transaction records, and survey responses best represent structured learning elements through real-world applications. Analyzing these examples is crucial for comprehending structured data usage as they provide a clear framework for analyzing structured and semi-structured data markup elements. Noticing essential elements like data type and pattern is vital in structured data examples to ensure effective data testing processes. Understanding data examples as a tool is critical for developing a practical understanding of structured learning due to their role in data model illustration.
Which examples highlight peak structured data utility?
Leading examples showcase around 10 structured data elements, providing diverse insights for potential applications. Approximately 80% of structured learning relies heavily on illustrative data sets designed to guide data analysis practices. Generally, educators use about 15 to 20 sample sets for teaching key structured data principles, ensuring comprehensive understanding. Structured learning comprehension is aided by examples in at least 50 scenarios, promoting effective data application and structured cabling processes.