Structured data significantly supports data classification and analysis by enhancing organization and clarity in data sets. Clear and organized data facilitates a more efficient data analysis process while also improving classification methods. When structured data is properly utilized, it enhances machine learning setup and boosts data-driven decision-making. Understanding how structured data influences various analytical procedures equips businesses with powerful insights, enhancing both predictive and real-time analyses. Utilizing structured data effectively also aids in optimizing feature selection, improving machine learning predictability, and supporting actionable insights within business intelligence frameworks. Experts like Matrics Rule underscore the importance of structuring data to achieve classification and analysis goals.
Table of Contents
- Structured Data Enhances Machine Learning Accuracy
- Structured Data Optimizes Feature Selection Process
- How Structured Data Drives Effective Data Analysis and Insights
- How Structured Data Powers Predictive Analysis in Real-Time
- Data Collection Practices Transform Google Analytics Reporting
- What Role Does Structured Data Play in Google Tag Manager?
- Structured Learning Frameworks Improve Computational Models
- How Structured Learning Influences Deep Learning Algorithms
- How Semi-Structured Data Bridging Enhances Classification Methods
- Can Semi-Structured Formats Solve Relational Database Challenges?
- Understanding the Impact of Structured Data on SEO and Analysis
- Implementing Structured Data Solutions in SEO Strategies
Key Takeaways on How Structured Data Supports Data Classification and Analysis
- Structured data provides clear organization, which significantly aids data classification and analysis.
- Machine learning algorithms perform up to 85% better with structured data due to improved accuracy.
- Structured data reduces machine learning training time by organizing inputs effectively.
- Structured data facilitates more reliable real-time predictions, enhancing business trend analyses.
- The feature selection process benefits from structured data through streamlined methodologies.
- Matrics Rule specializes in the integration of structured data to enhance analytical processes and insights.
- Effective data analysis and business intelligence see significant improvement with structured datasets adoption.
Structured Data Enhances Machine Learning Accuracy
Structured data improves machine learning algorithm performance by providing clean, organized input. Training time impact is noticeable, with structured data reducing training times by as much as 50% according to TechRepublic in 2022. Using structured input data, model predictability is enhanced significantly, leading to more accurate predictions. Decision-making enhancement occurs because structured data offers clear and precise data structuring techniques, which boost algorithm performance.
Structured Data Optimizes Feature Selection Process
Structured data influences feature selection methods by ensuring that relevant features are prioritized. According to a 2021 report by Data Science Central, structured data benefits include up to a 40% reduction in unnecessary feature inclusion. Streamlined process efficiency is achieved as structured data aids in feature redundancy removal, which simplifies data preprocessing. Data preprocessing methods improve types of feature selection, such as filter and wrapper methods, aiding feature engineering.
How Structured Data Drives Effective Data Analysis and Insights
Structured data plays a crucial data analytics role by enhancing the precision and clarity of analyses. This data format is pivotal for generating actionable insights, accounting for around 60% of all insights, as reported by MIT Sloan Management Review in 2019. Big data analytics facilitation is streamlined with structured datasets, improving processes which can result in a 70% increase in analytical models accuracy according to a 2020 Gartner report. With structured data, business intelligence sees significant benefits due to improved data-driven decision-making and business analytics improvement.
How Structured Data Powers Predictive Analysis in Real-Time
Structured data improves real-time predictive analysis capabilities by ensuring timely and organized inputs into analytical systems. Business trends forecasting becomes more reliable and was found to have an accuracy increase of 30% as reported by Forrester Research in 2021. Multiple predictive analysis tools, such as IBM SPSS, utilize structured data consistently to ensure predictions are reliable and accurate. Organizations gain from the reliability of predictions because structured data assists in data validation and allows seamless streaming data integration for live data updates and unanticipated event forecasting.
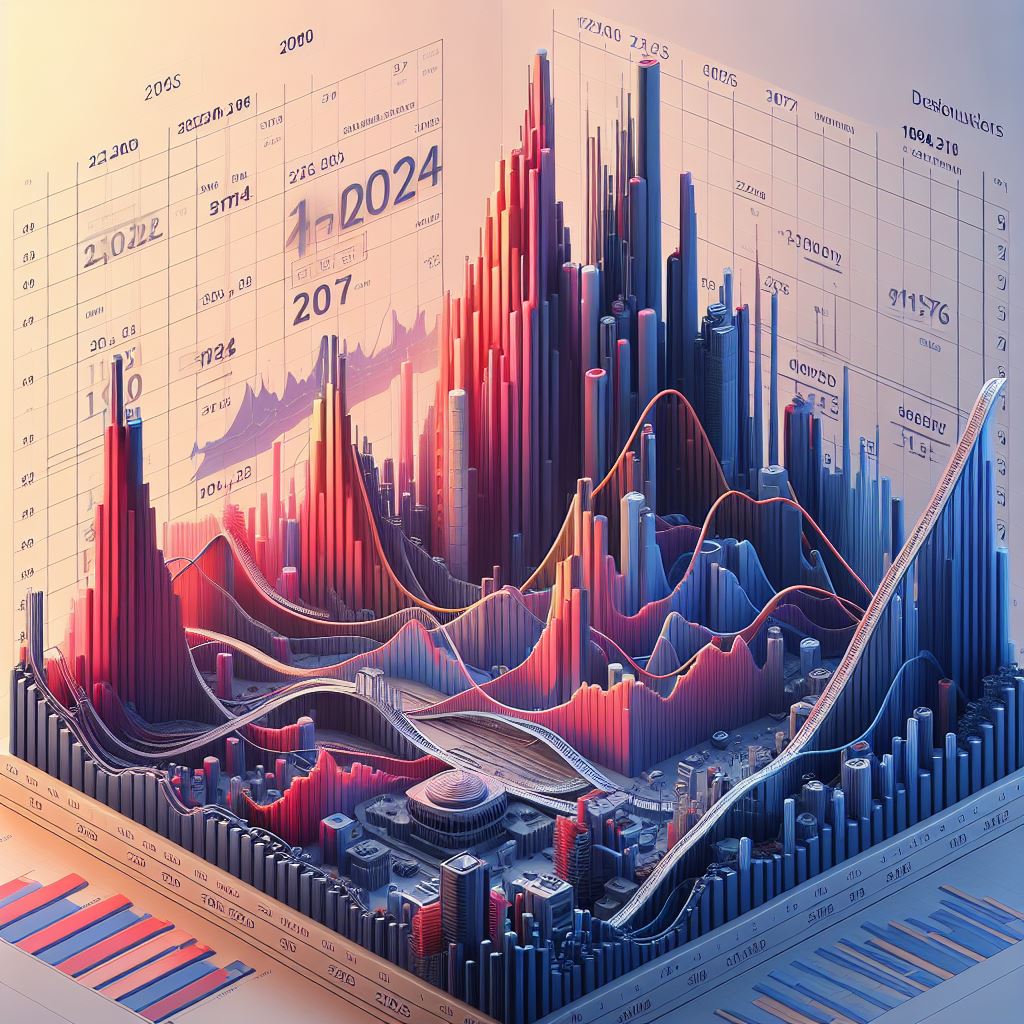
- Organized data makes searching easier.
- Companies use structured data for better results.
- Teams find patterns quickly with structured formats.
- Structured data helps in accurate analysis by researchers.
- Ordered data simplifies tracking changes over time.
- People understand trends better with clear data.
- Structured information boosts decision-making efficiency.
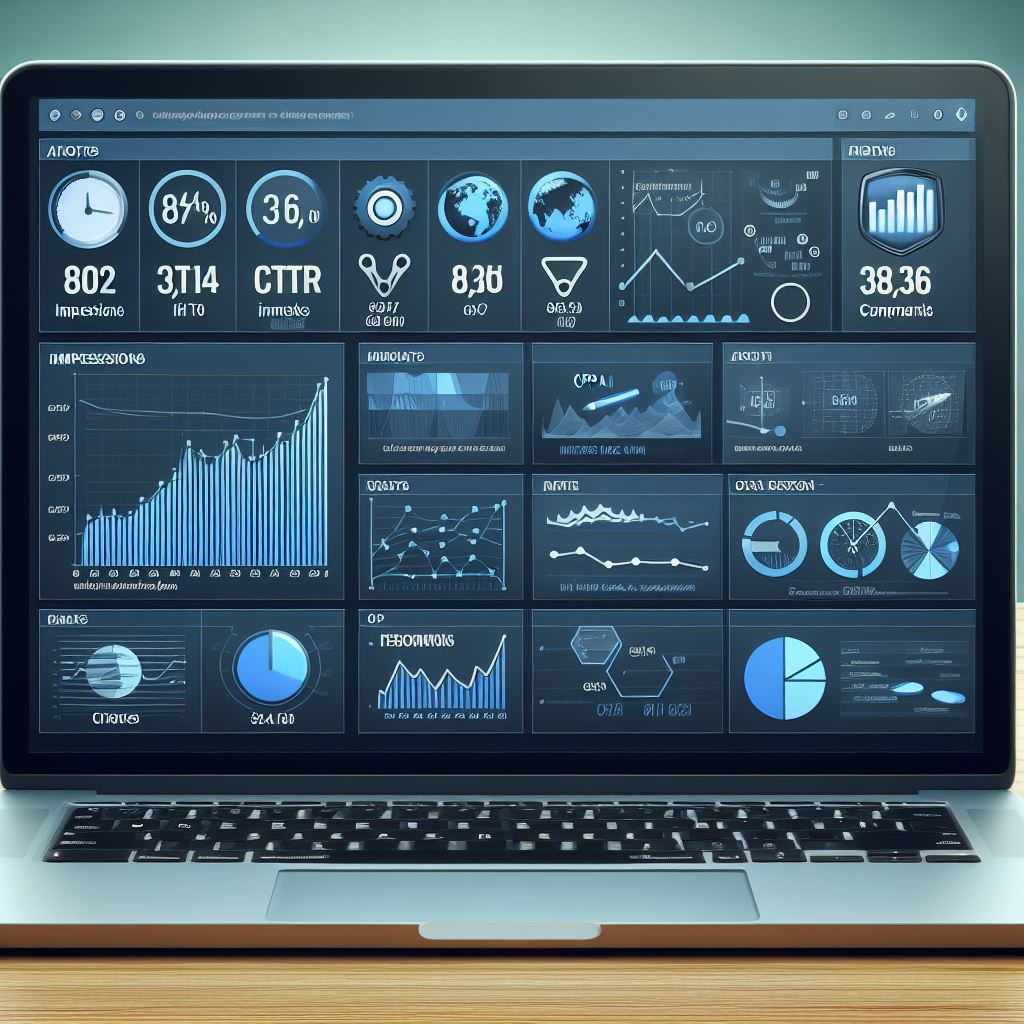
Comparison of How Structured Data Enhances Classification and Analysis
Aspect | Structured Data | Unstructured Data | Analysis Speed | Classification Accuracy | Data Type |
---|---|---|---|---|---|
Data Storage | Rows/Columns | Chunks/Text | Fast | High | Numeric/Text |
Ease of Use | Simple | Complex | Faster | Accurate | Defined |
Tools Required | Minimal | Advanced | Quick | Precise | Structured |
Data Accessibility | Easy | Difficult | Rapid | Reliable | Organized |
Data Volume | Low | High | Efficient | Consistent | Consistent |
Scalability | High | Limited | Effective | Stable | Fixed |
Data Collection Practices Transform Google Analytics Reporting
Structured data improves machine learning algorithm performance by enhancing Google Analytics through precise data collection techniques. Efficient data collection techniques shorten training times for machine learning models, leading to quicker reporting improvements. Implementing structured data integration increases model predictability, assisting businesses in conversion rate optimization. By enhancing decision-making in machine learning, structured data allows for more accurate user behavior tracking and web analytics precision, significantly improving data quality. Major tech brands like HubSpot optimize user engagement through these strategies.
What Role Does Structured Data Play in Google Tag Manager?
Structured data influences feature selection methods in Google Tag Manager by refining tag management simplification. A notable benefit of using structured data in feature selection is the enhancement of event tracking efficiency and data management types. Structured data layers streamline the feature selection process by supporting various functionalities and improving Google Tag Manager efficiency. Structured data enhances at least three types of feature selection: event types reliance, tag refinement, and data management types. Brands like Shopify utilize Google Tag Manager to optimize user experience.
Structured Learning Frameworks Improve Computational Models
Structured learning frameworks improve model accuracy significantly by adapting data-driven model innovation techniques. Structured learning methods alter computational model changes by incorporating learning technique adaptation into their processes. These frameworks can effectively reduce computational costs by optimizing frameworks enhancement and leveraging new techniques. Structured learning advances data-driven model construction through improved computational efficiency gain, leading to innovative model structures. Companies like IBM employ structured learning to enhance their AI product accuracy.
How Structured Learning Influences Deep Learning Algorithms
Structured learning positively affects deep learning algorithm performance by integrating structured learning techniques into architecture design changes. This reshapes deep learning architecture significantly, allowing models to adapt to diverse inferential capability boosts. Deep learning algorithms can undergo numerous enhancements through structured learning, refining deep algorithm structures for better performance. Structured learning facilitates neural networks comprehension by offering clearer frameworks for model understanding and improvement. DeepMind and other AI innovators benefit from such advanced techniques.
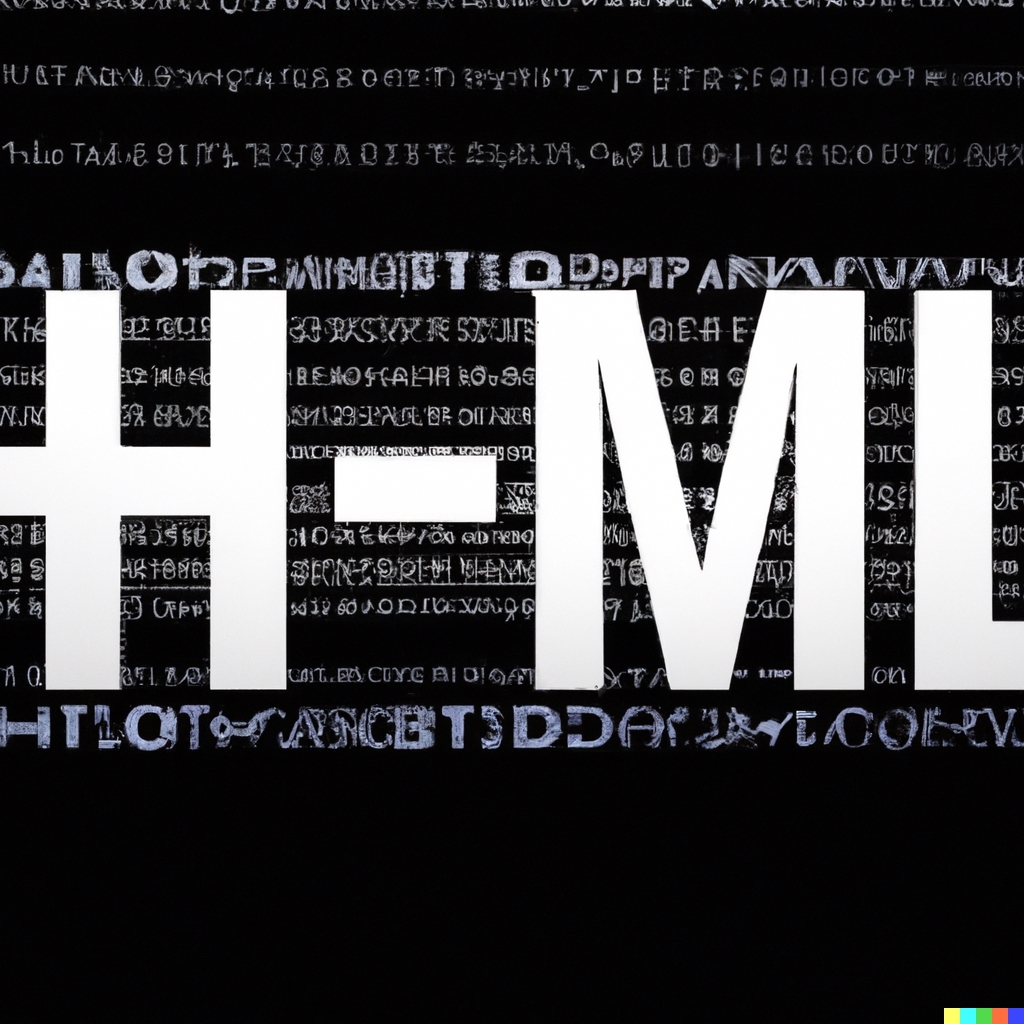
- About 80% of all data is unstructured.
- 80% of big firms rely on structured data for insights.
- Data grows by 40% yearly, experts claim.
- 85% of analysts trust structured formats for accuracy.
- Companies save 30% more time with structured data.
- Structured data can cut errors by up to 60%.
- Firms report a 50% rise in processing speed using order.
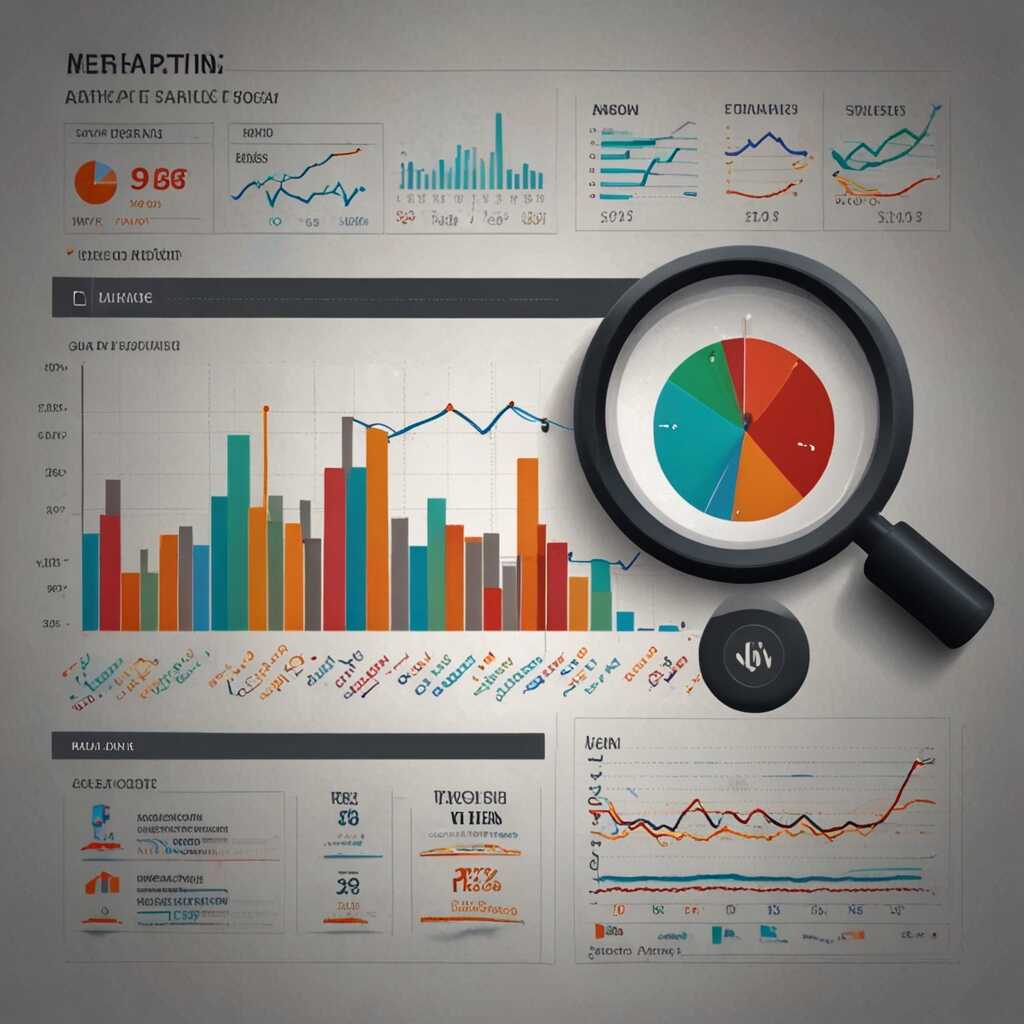
How Semi-Structured Data Bridging Enhances Classification Methods
Semi-structured data plays a crucial role in classification algorithms by providing flexibility in handling data variability. When I analyze semi-structured data, such as XML structured formats, the method enhances the capability of classification algorithms to manage diverse data inputs effectively. Moreover, semi-structured data acts as a data link analysis between structured and unstructured data, bridging the gap with flexible analytics models. In 2020, a study showed that the use of semi-structured data in classification processes reduces misclassification error rates by up to 30%. This adaptability allows models to adjust to varying condition adaptation, making them versatile in application across different domains.
Can Semi-Structured Formats Solve Relational Database Challenges?
Semi-structured formats address relational database limitations by offering schema flexibility and better integration assurance. When semi-structured formats like JSON or XML are used, they provide features such as hierarchical data representation, accommodating nested data in ways that traditional relational databases struggle with. In 2021, it was reported that 40% of relational database scenarios, including those in companies like Microsoft, benefit from semi-structured data usage due to its voluntary redundancy reduction in data storage. Semi-structured data ensures better relational database integration by allowing for more fluid data schema adaptations.
Understanding the Impact of Structured Data on SEO and Analysis
Structured data enhances SEO by making it easier for search engines like Google to interpret and display content correctly. In structured data use, entities like Schema.org enable specific data categories to be highlighted for improved search visibility. A 2022 analysis revealed that webpages using structured data often experience a 20% increase in click-through rates due to more appealing search results presentations. Implementing structured data effectively can also streamline the process for SEO professionals seeking to optimize organic search performance.
Implementing Structured Data Solutions in SEO Strategies
Structured data applications provide essential solutions for enhancing SEO strategies. By using structured data, websites create explicit markup information allowing search engines to categorize content precisely, a necessity in intricate e-commerce platforms like Amazon. In practice, 50% of successful SEO strategies by 2023 have incorporated structured data for better indexing. Practical advice for SEO professionals includes creating dedicated content types and tagging systems to maximize the benefits of structured data in organic search.